260 Leading Through The Disruptive Economics of Artificial Intelligence with Professor Ajay Agrawal | Partnering Leadership AI Global Thought Leader
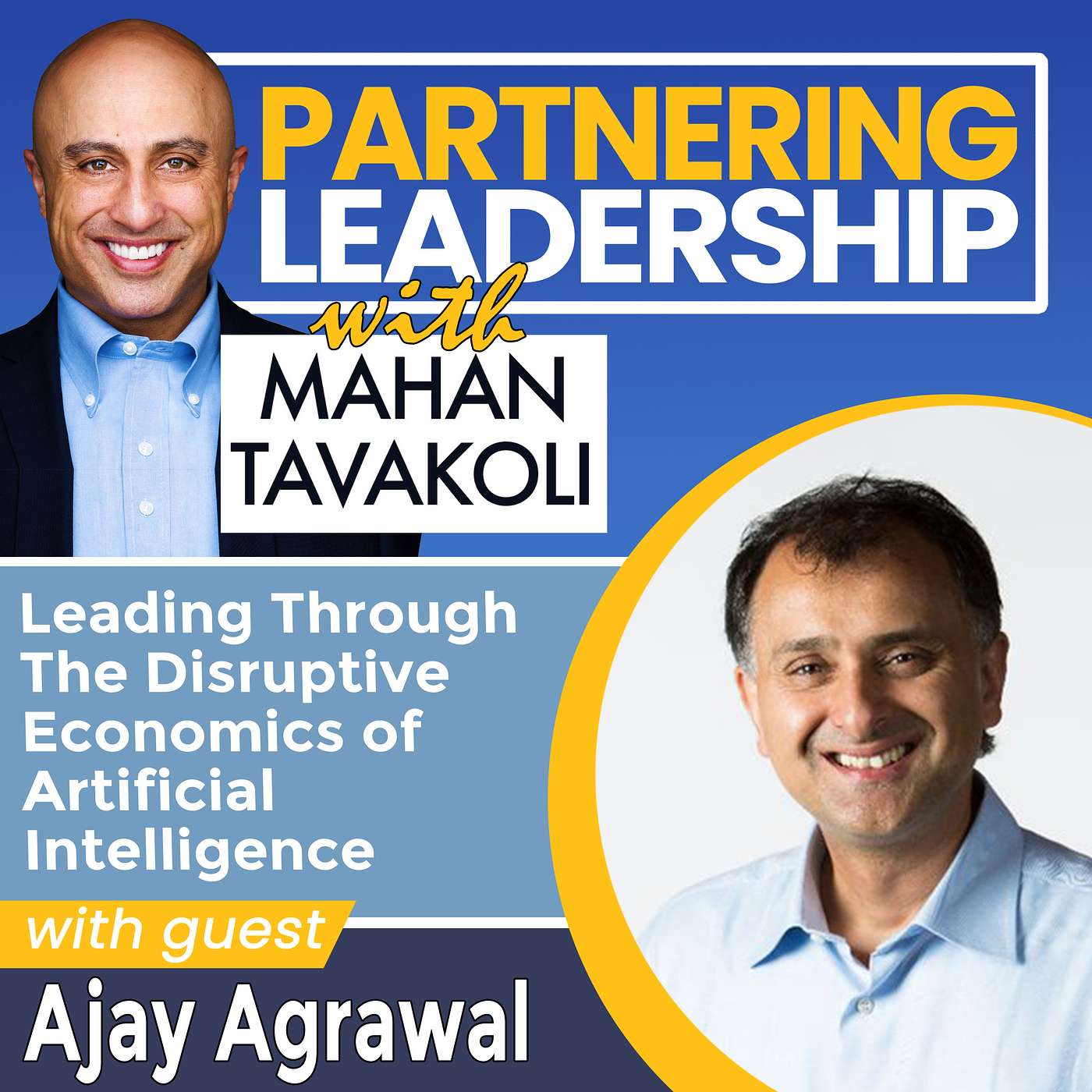
In this Partnering Leadership conversation, Mahan Tavakoli speaks with Professor Ajay Agrawal, the Geoffrey Taber Chair in Entrepreneurship and Innovation and Professor of Strategic Management at the University of Toronto's Rotman School of Management. Ajay Agrawal is also the founder of the Creative Destruction Lab (CDL), a not-for-profit program for early-stage, science-based companies, and coauthor of two outstanding books on Artificial Intelligence: Prediction Machines: The Simple Economics of Artificial Intelligence and Power and Prediction: The Disruptive Economics of Artificial Intelligence. In the conversation, Professor Agrawal shared the origin of his passion for studying the intersection of technology and economics and the increasing importance of artificial intelligence for organizations as a decision-making tool that brings down the cost of prediction. Ajay Agrawal then explained why this shift would significantly impact individuals, organizations, and industries as machines enable better predictions while humans focus on the judgment required for decision-making. Finally, Ajay Agrawal shared examples of the transformative impact of the reduction in the cost of prediction and how leaders can help guide their organizations through the significant changes ahead.
Some highlights:
- Three people that helped shape Ajay Agrawal's career path
- Why looking at AI from an economic perspective clarifies its potential to transform organizations and industries
- Ajay Agrawal on the role of predictions in artificial intelligence
- The importance of human judgment in decision making
- How AI decision-making will redefine roles in the workplace
- The disruptive economics of artificial intelligence
- How generative AI such as ChatGPT works and what causes mistakes and misstatements
- Ajay Agrawal on the importance of upskilling professionals
- How organizations can redesign their structures and processes to take into account the new predictive world
- The impact of AI on systems-level change
- How organizations can leverage AI for business success
- Why we're on the brink of a set of transformations that none of us have seen in our lifetimes
- How AI can help create a better future
Connect with Professor Ajay Agrawal:
Ajay Agrawal at Rotman School of Management
Prediction Machines: The Simple Economics of Artificial Intelligence on Amazon
Power and Prediction: The Disruptive Economics of Artificial Intelligence on Amazon
Connect with Mahan Tavakoli:
***DISCLAIMER: Please note that the following AI-generated transcript may not be 100% accurate and could contain misspellings or errors.***
[00:00:00] Mahan Tavakoli: AJ Agrawal, welcome to Partnering Leadership. I am thrilled to have you in this conversation with me. I was mentioning before we started recording, I started understanding AI first in reading prediction machines and in working with organiz.
I didn't see as much of a transformation as a result of ai. So then you wrote power and prediction the disruptive economics of artificial intelligence, answering my question. So I appreciate all of those. But before we get to that, would love to know, aj, whereabouts you grew up and how your upbringing impacted the kind of person you've.
[00:00:41] Ajay Agrawal: Sure. Mahan, thank you very much for inviting me to join you on your podcast and your interest in our work on the economics of machine intelligence. So your question is about my background and maybe three things from my background that are relevant to understanding how I think about this problem today.
One is I grew up with the plans to be an engineer my father is an immigrant from India and came to Canada to do a PhD in engineering at UBC in Vancouver. And he was studying Something called finite element analysis, which at that time was state of the art for understanding how buildings and structures like bridges and oil rigs and things react under dynamic loading, which it means like earthquakes and wind and things like that.
So when I went to college myself ubc I went into engineering and while I was there, I met three people that had a very significant impact on the trajectory of my life that would end up with, me doing the work I do now on economics of artificial intelligence.
So one was a professor named Hague Ferris Hague was a real anomaly on the UBC campus. He was the pioneer of venture capital investing in Canada. He started a company in the early seventies called Ventures West, and it was focused on innovations in the oil and gas. And mining. But he was interested in the technology part of those industries and in the founders the entrepreneurs like yourself
people who were creating, who had ideas and visions and would turn those into reality. He taught in the business school, but he made a deal with the business school dean that he would only teach he was a volunteer teacher. He donated all the money he got paid from teaching back to the university but he said he would do it under two conditions.
One is that he got a parking spot because very hard to park at ubc, they wanted a parking spot, and the other was he wanted half the seats in the c. To be offered to engineering students. In other words, normally all the seats are offered to only MBA students in business. He one and half the students to be engineering.
So I got a seat and it was all about the courses called the Formation Financing and Management of High Technology Companies. So that's where I started learning about the business. Of science and engineering. And then I had a second professor who was teaching economics.
So I took that class. It was a required class and I fell in love with the subject. It was the kind of class where you weren't supposed to fall in love with it because it wasn't an engineering class. It was a thing that you just had to do in order to get to the engineering part. I did okay in the midterm, but I didn't do great. And so I went to see the profess. After the midterm, and he was not that friendly to me and he assumed that I was there to try and negotiate a higher grade. And I told him like, I'm not here to negotiate a higher grade. I really don't care about the grade.
I'm very interested in the subject I just wanna learn from you about the things that I didn't get right on the exam. So I took then another economics course. And at this point now I had diverged in the first class it was full of my peers in engineering.
But the second and third class, I was the only one from engineering in those classes in economics, I took an economic history class and so on. And I realized that I was in love with this subject and I ended up carrying on and doing a PhD and all the time that I was doing work in economics, the area of economics that I was focused on was economics of innovation. The economics of science and technology, and then also the economics of entrepreneurship. So the underlying economics of, the people who are using science and technology to create. And then lastly the third person on my list is Persian. He is a graduate from a university in Iran called Sharif. And he was my roommate at university. I was born in Canada. But he had immigrated to Canada and he was interested in and doing work in something called payload monitoring. So he developed some very clever techniques that could be used in measuring the payload in excavating machines and mining while they were in motion without having to stop them. Which is a valuable problem in mining. When we both graduated, we were both doing our PhDs at the same time. I went off into Academ to get a professor job and he started a company. And he asked me to be his business advisor. So this was, two students one advising the other one.
He and I worked together on that and he built a very significant company out in Vancouver. So then fast forward to 20 11, 20 12. It's when I started getting interested in artificial intelligence and just to close the loop on those prior things with those three professors, the first one who taught a course on startups, I ended up starting two not-for-profit programs.
One called the next 36 that became Next Canada which is a national program to support founders. And the second called the Creative Destruction Lab. And Creative Destruction Lab is to my knowledge the largest. Program in the world of its kind. This year we took in 650 startups from around the world that are early stage science-based companies.
And hundreds of them are predicated on applications of artificial intelligence. Then with regards to the economics professor that I ended up. In my PhD, choosing economics, not engineering. And he had a very meaningful impact on my career and stayed in touch with him for many years.
Still do. And then the third two decades after he started that business, I came to him and said, I think you should look at these new developments in deep learning machine learning for what you are doing. With the company in robotics and mining. So he did and he put together a great team.
And then that company became the world leader in that application of artificial intelligence for machine vision in mining. And they've now become a British Columbia success story in what they're doing. That takes us up to present day and how I got to doing what I'm doing, which is, working as a professor of Applied Economics at the University of Toronto.
And the main initiative I have at the university is this program, the Creative Destruction Lab, which is focused on startups as well as next 36 and next Canada. And my personal focus area being on the economics of artificial.
[00:07:33] Mahan Tavakoli: Now, in the first book, prediction Machines, you talk about the importance of prediction in our daily lives. What role do predictions play in our lives? And then why would you state that artificial intelligence, in essence is a prediction machine?
[00:07:55] Ajay Agrawal: Sure. So maybe I'll answer your second question first the thing that economists are good. Is exactly the same thing that economists are bad at, which is economists are bad in a sense that they're not a lot of fun. Economists aren't a lot of fun to have at a dinner party because let's say you're talking about a new technology, most people will describe the new technology and revel in the magic of it and the excitement of it.
And an economist does exactly the opposite. They strip away all the fun of a technology and they reduce it down to a single. And that question is the same. It doesn't matter what the technology is. And the question is, what does this reduce the cost of? So for example in studying semiconductors, economists looked at semiconductors for a while and said, okay, we understand semiconductors lower the cost of arithmetic.
They make arithmetic cheap. Economists study the internet and then eventually say, okay, we understand internet lowers the cost of search. It lowers the cost of the digital distribution of goods and. Lowers the cost of communication. And same with ai. With ai, In some sense, it's probably the most magical technology of all.
When you see it in the movies in Hollywood, AI is what gives robots a soul and personality and it brings them to life. So people. Keep anthropomorphizing ai, they always compare. AI to humans and even the word ai, artificial intelligence is we don't call a washing machine intelligent, even though it's a machine that does.
Task for us. But when these computational statistics that underline machine learning do a task for us like a chat g b T, we keep using the words intelligence and then a anthropomorphize it. So as the AI world was starting to take off, 20 12, 20 13, 20 14. And because of Creative Destruction Lab, we got a very early front view seat.
One of the key pioneers of deep learning was a professor at our university, university of Toronto named Jeff Hinton and. He was subsequently, along with two others recognized with the touring award, which is like the Nobel Prize for computer science for this pioneering work.
And in 2012, that was the year that I launched the Creative Destruction Lab. One of his graduate students came into the Creative Destruction Lab and his name is Abe Heitz. And Abe showed up at the lab and he said, I have developed a new method. For drug discovery using this technique that my professor invented called deep learning.
At that point, none of us knew what he was talking about and he said this is a method that we can use for predicting which molecule will most effectively bind with which protein in a way that will allow us, rather than having to. Thousands of hours and many experiments in a lab trying to, figure out this binding efficacy.
We can predict it. So that was the first introduction , and at the time we thought, okay, this is some clever statistics. We didn't realize how powerful it was and how broad ranging it would be, but the more we started digging into it, the more we saw what the range. And in the very beginning, at the way I just described to you, I used the word predict, but at that time people weren't using the word predict.
They were saying, oh, we can generate these binding efficacy numbers. And somebody else was using it for reading. Medical images in radiology. And somebody else was using it for doing fraud detection at banks. So we were looking at all this, and then we realized, wait a minute, these are all using the same statistical tools, machine learning, and what is the common link between these and what are these machine learning algorithms actually doing?
And I think which seems simple now, but at the time, to us was an insight was, wait a minute, this is all just prediction it gives the sense of being human-like, but it is just prediction and what machine learning is doing. Advances in machine learning are lowering the cost of prediction and the minute that sentence came out of our mouth that the rise of AI is actually a drop in the cost of predict.
Then the light bulbs went off because we said, wait a minute, we've got all these tools from economics. As soon as we say it's a drop in the cost of prediction, downward sloping demand curves that as the cost of something falls, the demand for that thing increases. And then we know well that will.
Increase the value of compliments to that thing, it will decrease the value of substitutes to that thing that all these pieces started snapping into place. And so he said, wait a minute. This is such a foundational technology that does such a foundational thing, prediction that we should really think hard about what are the economic implications of this?
Because now we put it into a language that was the core of what we could build all the other economic pieces. So to answer your second question that's why we focused and. The language we use around AI is dropping the cost of prediction and that's what AI is.
It is just, computational statistic that does prediction. And even though it doesn't look like it like when you do chat g p t and you type in your thing and it produces language. We don't think of language as the outcome of prediction, but that's all it is predicting what they call tokens.
And a large language model like chat, G P T, is a token prediction. And if you look at for example a driverless car that's an AI that's making predictions and all it's doing is it's getting all the input data from the camera, the radar, the lidar, the various sensors on the outside of the car, and.
It's AI has learned to predict what would a human do under these circumstances. So it gets these, hundreds of thou, millions of hours of human training data so that it can say, okay, when I see this thing, when I see this red sign when I see these types of lines on the pavement, when I see a child approaching the crosswalk I can predict what a human would do under those circumstances when you and I learn language, or let's say in your case where you learn the rules between going from, let's say Farsi to English or English to French, you and I learn them as the rules between going between these language and then the exceptions to the rules.
But an AI translate, like Google Translate doesn't learn. We feed it millions of pairs of professionally translated documents from like the United Nations or multinational corporations. So that. It can predict. Then after it gets enough examples, it can predict, if I see a sentence like this in English, what will it look like in French?
Or what will it look like in Farsi, even if it's never seen that English sentence before prediction. So the answer to your first question is that's why we focus on prediction because at his core, that's what it is. And it's so clarifying for people because otherwise they look at chat G B T and they look at a driverless car and they look at machine reading.
MRIs in a hospital and they don't know what they all have in common. And then as soon as they realize at the core of all of these, it's computational statistics, producing predictions, then it makes it so much more manageable for people to understand how it's working, but most importantly, what are its strengths and weakness.
When chat g p t spits out a sentence that's inaccurate or it makes up something that sounds real, but is false. You say, wait a minute, is chat gpi trying to lie to me? No. Now you understand what it's doing is it's predicting what words or set of words would come next after this based on all the Texas read, from the internet up to the end of 2021 and it has no.
It has no logic in its brain. This is what is the most likely thing set of words to come out given this prompt. And so it's not trying to lie to you, it is just drawing from a probability distribution of what is a likely response.
[00:16:22] Mahan Tavakoli: Aj that. Power of prediction helps us and has helped me understand AI. Now, as I think about it in different types of roles. First before the many opportunities, it could open up. A lot of roles are primarily prediction dependent. For example, doctors to a great extent.
Prediction machines. So how will this AI, and how will the drop in cost that you talk about impact all of these roles that if we think about are to a great extent, prediction machines in a human brain.
[00:17:13] Ajay Agrawal: Yes, this is a great question. First of all, when you say many roles, I would come back to you and say all roles.
Because any role that has a decision or a set of decisions , when you make a decision. All of us, when we make decisions, there are two things happening in our cognition. And because they happen together we don't even think, most people never think of them as two separate things.
We think of them as just one thing, which is, oh, I had a thought, or, oh, I made a decision. But what's happening is actually two things. One part is prediction and one part's judgment. The prediction part is in the face of uncertainty. What's the likelihood of different outcomes? And the judgment part is what's the consequence of that outcome.
In other words, how good or bad is that consequence? And so one is a prediction of the probability of that consequence, and the other is the benefit or the magnitude of the benefit of the cost of that. That's the judgment part. And so what machine intelligence allows us to do is to offload the prediction part that we normally do in our brain, and we do it together with a judgment, and they're so tightly coupled that we don't even think of those two things.
It allows us to offload that and give that to a machine. So now we're just doing the. And so that will lead to, in the longer term a redefining of roles. So rather than talking about a role it will be a full scale redefining of roles. And many people compare this to, for example, the mechanization.
Agriculture, that there was a time where over 50% of the population worked in agriculture. Think of all the many different types of jobs that there were in agriculture, and then if we talked about, okay, this industry's now gonna get mechanized, how will that change our roles? Nobody would've said, oh, it's gonna change A farmer who.
This particular thing into a video game designer it just, it doesn't even compute. It's not the same thing at all. It's not like that is modifying that role. That entire category of work has been mechanized and now it's left people to pursue different things. And so let me give you one example. So the example is an AI that we use for navigation, like Google Maps or Ways or something.
So that's an AI that predicts the optimal route between point A and point. Now, one piece of background that you probably know from reading the books, but maybe some of your listeners don't know, is that if you want to drive a taxi in the city of London, you have to go to school for three years to learn what they call the knowledge.
And so there's a number of small schools around the city of London that offer, this training is three years and the people spend the first year studying maps of London. The second year they get on mopeds and they ride around the city and they're learning the city that way. And the third year they do a combination of maps in mopeds, and then at the end they take an exam.
And the exam will ask questions like you pick up a passenger four o'clock in the afternoon, on a Thursday in November, and the passengers at the Churchill War rooms, and they want to go to the Royal Botanical Garden. What's the fastest route? And so they give the answer and they're given a bunch of questions like that and they give the answers.
Now today, of course, you and I could fly into Heathrow Airport, rent a car, and we've never been to the city of London, and we open up our navigational. And we can navigate the city as well as a professional who's had three years of training. Okay, so we get this AI and. What would we do with it? Now it turns out in driving there's actually two predictions which came as a surprise to me.
I was actually thinking there's one, which is what's the prediction of the best route? But it turns out there's a second key prediction in taxi driving, which is the first prediction of the best route is what you do when you have a passenger in your vehicle. But the minute they get out of your vehicle, there's a second prediction, which is, where do I drive my.
To minimize the time before I catch my next ride. And so there's a group of economists that got access to someone who developed that second prediction machine and ai, they called it Navi ai. And it was to predict where a driver should go after they dropped off their
[00:22:17] video1681534547: passenger
[00:22:18] Ajay Agrawal: to minimize the time before they pick up their next p.
And what they did is they gave it to a bunch of drivers. And then others they didn't give it to. So some drivers got this Navi ai, some drivers didn't. And then what they did is they measured the productivity of those drivers and they measured it for everybody before anyone got Navi.
And then they looked at the change. For the ones who got nav ai, how much more productive were they afterwards comparing it to the control group that never got it. And they measured the productivity before versus after. And the treated versus the control group. Okay. And here's what they found was that the drivers who were new on the job, what they called inexperienced drivers, They got a 7% productivity lift from this ai.
The experience drivers got zero lift. They already had a very good instinct of where to go. Now, you could imagine if you had built these ais, Like navi, ai and navigational ai like ways or Google maps or something. And you wanted to start a business, you might decide, I wanna license this technology and my customers will be enterprise and the most likely industry will be taxi industry because, they're all the time have to go new routes and.
This would be a good tool for them. And it gives the less experienced drivers a 7% boost. The more experienced drivers zero boost. So some companies would license it, some would say, no, we don't need it. And the ones who license it would, pay a small licensing fee to give their newer drivers a 7% boost.
So that would've been the business model if we had just done the normal type of business of these ais. There's another business model that would've been a point solution, and that was what power and prediction's about and how you opened this segment about why haven't we had such a big impact?
Almost all the applications we've seen of AI so far have been of that type point solutions. So for example, in banks use AI for fraud detection, to predict which transactions are fraudulent. And so what they do is they already had predictive analytics to do fraud detection.
They take out those old analytics, they put in the new ai, they get higher fidelity predictions of fraud. But the rest of the bank process stays the same. That's why we call it a point solution. The system stays the same and they surgically go in, take out the old prediction, drop in the new one, but the rest stays the same.
Same as if we were to give the taxi drivers a navigational AI tool, and it makes those taxi drivers a little more productive, but the rest of the taxi system stays the same point solution. Now what we got to see, Was a system solution and that was Uber. So that was, instead of making professionals 7% better, we gave it to everybody.
And now, for example, in the United States, before Uber, there were 200,000 registered taxi and limousine drivers, 200,000 drivers. In the US today there are between three and 4 million people who drive for. And each one of them, let's say on average is bringing a car onto the road. Let's say there's $25,000 a car on average.
That's a hundred billion of underutilized CapEx that was sitting in people's driveways and car parks that are now brought into the transportation system to move people around the cities.
So this is one example where now people did not need three years of training to drive in the city of London professionally. In other words if without a navigational ai, if Uber had tried that without a navigational AI and you got into some random person's car and you told 'em you wanted to go to this place, and three minutes into the driving, they pulled over and had to get out a map and figure out how to get there, you would never do that.
You would say, I'm gonna stick to the taxi drivers, the pros. But because now anyone can navigate as well as a professional, it completely transformed the industry. Now imagine that across many industries. In our country we have people that are lined up outside, hospitals for example, waiting for this test or that test and to get medical attention for this and that. And imagine a scenario where many more people are able to do certain functions because they are UPS skill.
The way , anyone who had the license to drive a vehicle was now upskilled to navigate like a pro. Now think of all the other domains where people can be upskilled, and navigate. And so that is coming. That is coming and for anyone who's listening, who doesn't believe it, think about how.
A person who wants to write an email that now has chat, G p t, who is upskilled. So for example, a few days after chat, g p T came out, I saw on Twitter a doctor post a note, and this doctor \ was writing a letter. On behalf of one of their patients to an insurance company so this doctor had said that his patient needed to get a test and the insurance company had rejected that patient that they would not pay for this test.
They said it wasn't necessary. And so now the patient had come back and the doctor had to write an appeal letter and so the doctor, instead of writing the letter, just wrote two bullet points about the patient and their condit. And instructed chat g p t to write the letter. And then he posted the two bullet point prompt, and then the letter that chat g p t produced on Twitter for all his doctor friends to see.
And then you could read all the comments of how good the letter was as evaluated by the other doctors. The reaction was we spend so much time writing letters to insurance companies, that is time that we could otherwise be spending with. All these doctors were saying, , this is gonna transform how I conduct my practice.
And that was about five days after chat g p t was out there, still all kinds of problems and bugs and the rest of it. So just think what's coming. I think most people have no idea. All of us saw what happened with. Largely, despite all the negative things that people have said, and many of which are very legitimate about the nature of the founding CEO of Uber Travis Kalanick.
He operated in the gray area of the law in the entry of many cities and so on and broke the law in a number of cases. But what he did was he demonstrated to the world what a system levels change looks like. And so to everybody who now thinks that transportation in America is measurably better than before, You may not like the tactics that he followed, but he gave us exhibit A to demonstrate what can be done in our education system, in our healthcare system, in our insurance system.
Everywhere. And so we haven't done it in those areas. Even though we have ais that can read pathology slides better than experienced docs. We have ais that are better able to read medical images than radiologists. We have ais that are better able to predict attempted suicides. The mental health experts.
We still haven't brought these into clinical practice at large scale because of all these barrier. The same kinds of barriers that Uber kicked down in the taxi industry are preventing the deploy of system level change in these other sectors. And many of it for good reason, like to protect people, to protect jobs, but also many for bad reasons, which is to protect the status quo.
And things that in the long term are harming society. So there's a lot coming. I think. We're on the brink of a set of transformations that none of us have seen in our lifetimes unless for those that, participate in the second World War.
I,
[00:30:43] Mahan Tavakoli: Really appreciate that response, rj, and I totally agree with the sentiments. I am also thrilled and excited about the potential for everyone to be upskilled and what you talk about, system level change, and would love to. Touch on that. Will system level changes come from new entrants to the market?
[00:31:04] Ajay Agrawal: They can certainly come from anywhere in some respect. Existing organizations have a head start. They already have customers, they have users, they have reputation, they have all the things that give them an advantage over startups.
The reason that they often struggle with doing a system level change is because they are totally designed and organiz. For a pre prediction world. So the way to think about it is think about every organization, whether it's for-profit like a company or not-for-profit like a hospital or at least some hospitals or government agency.
Every organization is optimized to achieve whatever its goal is, and that optimization has. In a pre prediction world. So it's being optimized, given the tools that were available, to it at the time it was created. So now there's this shock hits the world where a new thing is able to do very high fidelity predictions much greater than what we've had before.
And you've got an organization that its entire structure. Has been built for a world before that existed. So it's very hard that's why everyone has focused on point solutions, because that's the thing where it said, okay, no problem. We can just pull out our old predictive analytics and drop in the new ai.
And that's why all the existing organizations have followed that method.
But it's very hard to say, okay, we're gonna start from scratch and redesign. Like, how would our organization look if we were to design it today in a post prediction world where all these things that we have assumed, like if I point to you Mahan a large warehouse, I would tell you that w.
Is a monument to uncertainty. It exists because we don't have good prediction. If we had good prediction, we wouldn't need the warehouse because the warehouse is there. Because we can't predict, we don't know what these people are gonna want that live in this neighborhood in dc And so we put a warehouse in DC where we can put all the stuff that we think people might.
And then when the actual retailer says, oh yeah, there's a bunch of people in this neighborhood that want this thing, then they pull it outta the warehouse and take it to that retail location. So a warehouse is a monument to uncertainty airports. Think of how crazy airports are that they've become this huge structures with fancy, shopping and all this stuff.
Airports are not for shopping. Airports are to go to a. And here's the craziest thing is that you go to these fancy airports and they've got Prada and Gucci and all the fancy stuff for regular people like you and me. If you go to the wealthiest people in the world, they don't go to those air airports cause they fly private and you go to the terminal at a private.
Airport. There's no Gucci, there's no Prada. So how come regular guys like you? Me, we go to the airports with the Gucci and the Prada and the very wealthy people, they don't get Gucci in Prada because they don't have to wait because an airport, it's a monument to uncertainty. We build these big infrastructures because people go there and then have hours of time to.
Because they don't know exactly when they need to be there in order to get on their plane. If we knew perfectly what time we had to leave our house in order to walk right onto the plane, then we would just leave then and we would leave and we would drive up and we would walk through security and we'd get to our gate and off we'd go.
But all that infrastructure's there because of uncertainty. And the reason that the wealthiest people don't have it is because they don't deal with the unc. These things are going to be redesigned. And in terms of your question, is it gonna be startups or is it gonna be existing companies?
If the existing ones have the stomach to make the system level change, then it will be them. But as we saw with the taxi c. It's so hard for them to change. Even when the taxi company saw what Uber was doing, it was like a slow motion car crash. They saw it, they couldn't react, and so instead they went running to the regulators and said, please protect us.
These guys are breaking this rule and that rule. Meanwhile, customers were like, but there's so much better. It's so much better. And the taxi companies could go to the customers and say, Hey, please be loyal to us, because not all of them, many of them had been providing such terrible service that their only recourse was to go to the regulators
[00:36:04] Mahan Tavakoli: AJ for that system level change, I've seen AI centralized decision making. So in essence, take judgment out of the hands of individuals involved. So AI that is used for hiring processes in larger organizations is taking that judgment away from.
The frontline manager who used to play a role or their judgment would play a much bigger role in the hiring decision. How can we think about this centralization and what impact will that have on organizations and on society at large as more of these decisions are being centralized through ai?
[00:36:50] Ajay Agrawal: I think there are two parts to what you said there. One was central. And one was automation. And I think those are two different things. So automation would be person applies, machine reads their application and the machine makes a decision.
In our book, we have a chapter that's called Machines Don't Decide. And so even if it looks like a machine is deciding, all that means is a human has coded their judgment into the software. So the AI makes a prediction and then the software references the judgment that has been baked into the software that some human has put there and said, okay, I'm taking the prediction that I just made and the judgment that's been given to.
In advance, it's now baked into the software, and then I will execute on that. So it looks like the machine made a decision, but really the judgment has just been put there by the whoever made the program. And so the question Is not, does hiring require human judgment? Whether a machine delivers what looks like the decision or a human does, a human is still delivering the judgment.
It's just whether they do it in real time or whether they've done it in advance and put it into the software. And all the good HR systems that I've seen that use ai, use it as a recommendation engine. So it's still a human making the judgment, but it's being recommended. And now centralization is that. A lot of decisions that might have been dispersed, let's say, around a bunch of different field offices.
All that data can now be centralized and processed. And so you can have a small group, let's say at the headquarters who's getting it, and they might be getting an AI recommendation, and then they're making the judgment there. Or for example, let's say in a military setting where you have a drone that's, in some war zone.
And then it's sending a recommendation of whether to fire to Washington and someone there is making the decision. So that looks like it's centralized. So rather than, for example, a human soldier on the ground making that decision, which would be distributed decision making, now there's a drone and there's a centralized person in Washington deciding.
So there might be five centralized people deciding rather than, 5,000 soldiers on the. Yes, it can be used for that kind of centralization. And we have to decide as a society or as companies, depending on what the nature of the decision is whether we think it's important that the judgment be delivered at the time of the decision, or if it can be deliver.
Xi in advance and put into the software, and I think those are the types of regulatory discussions we should be having. In my view we should really be staffing up in places like Washington and Ottawa and Canada and in Geneva. In places where people are setting policy in order to dig deeply down into the issue of judgment for a very wide range of decisions.
Like right now we have a body like the FDA that regulates which drugs we are allowed to put out into the. Because there's a lot of uncertainty around the drugs some drugs have worse side effects for some people than other people. And so we test the drugs in a wide range of settings for children, for adults, for males, for females, we do all these tests and then we measure the likelihood, what's the likelihood, the probability of this kind of a side effect. And what's the cost of that side effect relative to the. So we have a body for doing that. For drugs. We've effectively need an FDA for AI driven decision making.
And what that would do is it would put different types of decisions into different categories and say, okay, for this category, these are the rules around how we deliver judgment and how we handle predictions. Like, how confident do the predictions need to be? What's the error rate that we are gonna allow for both false positive and false negatives for these types of predictions in this category.
So that's how Mohan, I would be thinking about.
[00:41:00] Mahan Tavakoli: And that's why it's really important, rj, I appreciate you mentioning that. And it's important for organizational leaders to read and understand prediction machines and power and prediction to understand the potential system level changes in their organizations.
But also, I have a lot of. Both local and national policymakers listening. This is really important. The potential is huge, but we have to have those kinds of conversations ahead of time in order to be able to take advantage of the opportunities. I know we touched just on the very surface of your insights, aj.
How can the audience find out more about you and your books?
[00:41:44] Ajay Agrawal: They can just come to my website. I have a personal website and just Google my name, a first name, a j a y, last name Aggarwal, A G R A W A l. And then my website is just aggarwal.ca. CAS for Canada.
[00:41:56] Mahan Tavakoli: I really appreciate this aj.
You also mentioned to fully capitalize on its potential organizations need to invest in the right talent, infrastructure, and data capabilities. They must be willing to rethink their business processes and strategies to leverage AI effectively. So thank you for helping organizational leaders understand how to implement AI effectively.
And thank you for challeng. Us and the policy makers to think about the potential and taking advantage of it. Really appreciate the conversation and your wonderful books and insights. Thank you so much, AJ Agrawal.
[00:42:35] Ajay Agrawal: Thank you very much. Thanks for inviting me and thank you for your excellent questions.