264 AI Ethics, Leadership & Governance with Renee Cummings, Professor of Practice in Data Science at UVA | Partnering Leadership AI Global Thought Leader
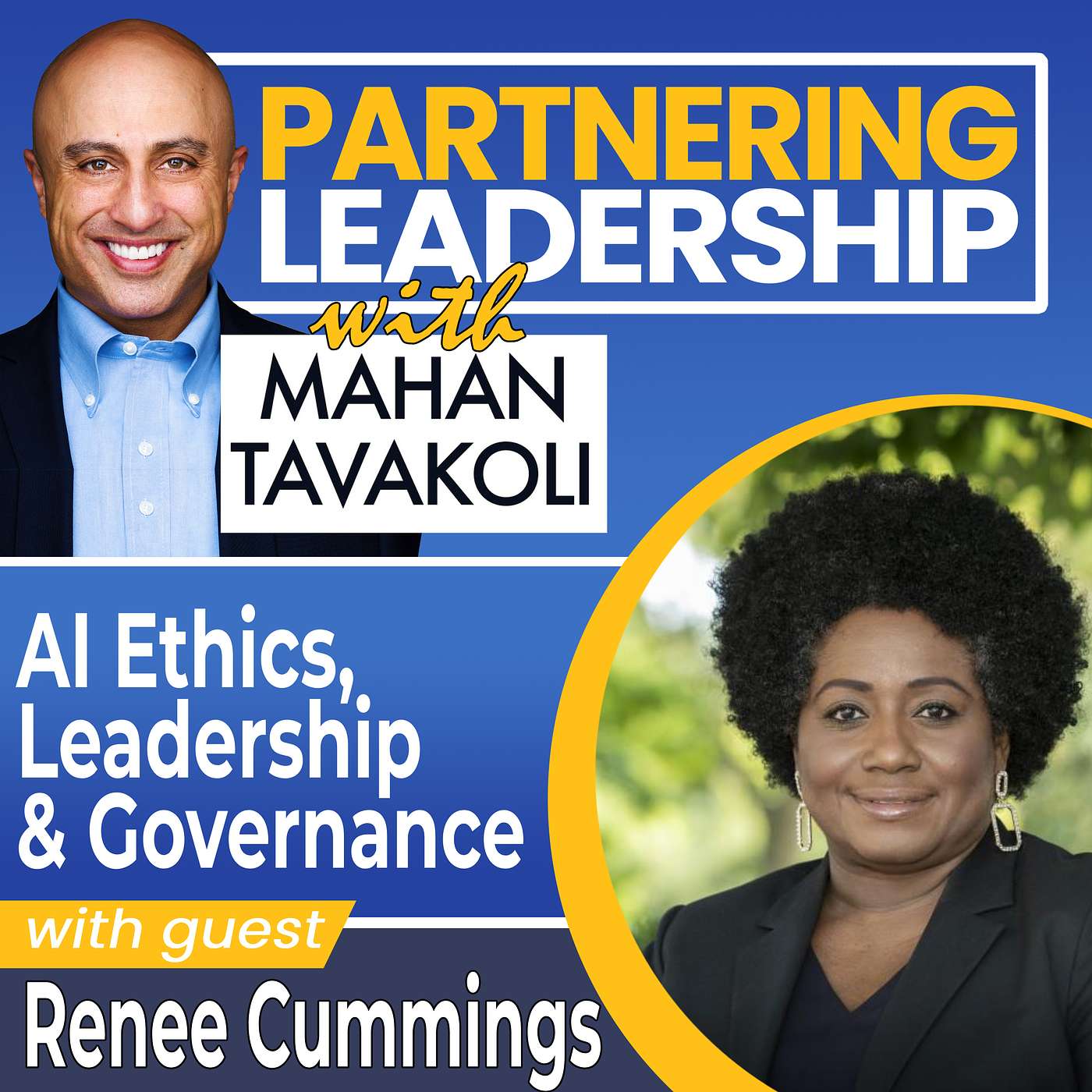
In this episode of Partnering Leadership, Mahan Tavakoli speaks with Renee Cummings, Data Science Professor of Practice at the University of Virginia. In the conversation, Professor Renee Cummings, a criminologist and AI ethicist discusses the importance of bringing responsible, ethical thinking to deploying AI technology. She stresses the need for due process, recognizing the various forms of bias, ethical use and transparency in deployment, and good governance and oversight. Renee Cummings also addresses the need for considering diversity, equity, and inclusion in AI development and deployment and how we can ensure that AI benefits society instead of harming it.
Some highlights:
- Why diversity of experience and perspective is crucial in AI
- The role that data plays in AI and data as a store of both positive and negative aspects of humanity.
differently, and the difference that makes in AI development and deployment
- Renee Cummings on the role AI has played in law enforcement for the past 20-plus years
- Why it's important to keep humans involved in the decision-making loop rather than allowing extreme decision-making autonomy for AI systems
- How to design technology ethically to avoid extreme prejudice and further persecution of certain groups
- Professor Renee Cummings on the many opportunities created by AI
Connect with Renee Cummings
Connect with Mahan Tavakoli:
***DISCLAIMER: Please note that the following AI-generated transcript may not be 100% accurate and could contain misspellings or errors.***
[00:00:00] Mahan Tavakoli: Professor Renee Cummings, welcome to Partnering Leadership. I am thrilled to have you in this conversation with me.
[00:00:06] Renee Cummings: Let me tell you, it is such an honor and a very unique pleasure to be joining you in this conversation. I look forward to with.
[00:00:14] Mahan Tavakoli: Renee, I am so excited having studied some of the work that you've done, so I'm very fascinated both with your background as a crimin.
And now as a data science professor deep into AI and ethical ai. But before we get to that, would love to know whereabouts you grew up and how your upbringing impacted the kind of person you've become. Renee.
[00:00:38] Renee Cummings: Fantastic. Question. So I was born in Trinidad and Tobago in the Caribbean, and growing up on an island that is so cosmopolitan diverse and so inclusive, really shaped my thinking.
So I bring a very multicultural worldview to the work that I do. I grew up in a land that is just so rich with culture and creativity and energy and the way that we do things in Trinidad and Tobago. The world has always celebrated us. So we're a very small island with some very big achievements.
Of course, we've produced many scholars. Including our first prime Minister, Dr. Eric Williams, of course, the scholar, c l r James, the father of the pan-Africanist movement George Patmore we vs. Nial who is one of The great writers of the world and of course a son of the soil and of course Nobel laureate Derek Walcott, who although he was born in Salia, considered Trinidad and Tobago his home.
So we have this richness that we bring and a bigness that we bring. Although we're a very small space, so I think that contributed to who I am. I'm also a mix of various cultures and heritages. My grandfather is of Indian descent, what we people consider Southern Asian. So I grew up in a household with African influences, Spanish and French, and of course Indian influences.
I think all of that makes me who I am. And that is the energy of diversity and inclusivity and just equity that I bring to this space of technology. And this really broad perspective, interdisciplinary, sort of multicultural perspective as.
[00:02:22] Mahan Tavakoli: What an outstanding background, Renee, and you beam with pride.
As you talk about all of the people that have made Trinidad and Tobago proud. You can add to that list. Renee Cummings.
[00:02:38] Renee Cummings: Thank you very much.
[00:02:40] Mahan Tavakoli: You have also achieved a lot, done a lot, and you are a significant voice. Something that I believe will transform all of our lives, more so than any of the technologies that many people are comparing to, and that's artificial intelligence.
But Renee, before getting involved into data science and ai, you became a criminologist. Where did that interest come from?
[00:03:08] Renee Cummings: I was actually working as a substance abuse therapist at a therapeutic community in New York City trying to provide rehabilitation interventions and a change lifestyle, the persons who were arrested because of substance abuse.
While I was there, I realized that all of my. Clients had a criminal history and I was really interested in the relationship between crime and substance abuse. And that led me to the John Jay college of Criminal Justice in New York City. And while I was there and.
Studying crime and criminality and drugs. I looked at other questions such as juvenile delinquency, juvenile justice forensic psychology, and even terrorism studies. And I got a master's degree in terrorism studies because of course I was always interested in the mind. The mind of the perpetrator, why individuals perpetrator a particular crime, the behavior, the crime scene.
So I think all of this really added to the diversity that I bring into the space of data science technology and of course artificial intelligence, which is my new love as I call.
[00:04:12] Mahan Tavakoli: Renee, our experiences in life shape the way we see the world. And one of the challenges with a lot of technologies has been the people that have started the companies and shaped the world have had.
Singular experiences. So one of the challenges that I see with some of the social media geniuses and people that made it so successful and became so rich as a result, is that they had very similar backgrounds, very, in my view, High iq, questionable eq. That contributed to some of the negative impacts that all these years later were finding about social media.
So you have that unique experience now. A lot of the people who are starting up AI companies are people that have very similar backgrounds to the folks that started the social media companies. How can we make sure that there is more diversity of experience and perspective as these companies are?
[00:05:30] Renee Cummings: I think we've gotta think about the individual. We've gotta think about people, and no one is just one thing. We're all sum total of all the experiences that we've had. There's diversity in the things that we like. The things that we eat, the things that we do, the company that we share the kinds of experiences that we find to be wholesome, that really contribute to our wellness.
And our wellbeing. The people who we talk to, the people who we hang out with, the kinds of places we go to die. And I think this is what makes us who we are. We are just not this one thing, this monolith. We are so much more than just. And I think for me, I have always been in careers that focused on people and that diversity, and I think what continues to drive me from my work to criminology into data science and now into responsible technology.
Is justice because from I come from a place where justice is important, and justice is what rules this world. Justice is what keeps us, together. It keeps us thriving together. It keeps us building together. And it's not only criminal justice, it's environmental justice. It's food justice now it's data justice.
And it comes back to questions around equity, questions around inclusion, questions around diversity. All of these things contribute to justice. So when we talk about justice, we're speaking about fairness. We're speaking about the ways in which we could ensure that all of us are able to enjoy this technology, the way we can use this technology to benefit humanity.
And if you use something in a just. If you focus on fairness, then on and due process, and you bring the requisite level of due diligence and you understand that we've got to build technology and deploy technology with a duty of care an ethic of care as well, and we bring these ethical perspectives.
Then we know we are doing things in a way that's going to lift humanity up, advance humanity, and solve some of those challenges that we have never been able to solve. But if we do it in a way that is a limited sort of myopic approach, then we're undermining the creativity of the technology. I always say that AI.
Really excites me because it has the opportunity to do these extraordinary things to transform every business model, to transform everything we do from the ways in which we communicate with each other, the ways in which we work, the ways in which we solve problems, the ways in which we improve our health, the ways in which we live longer and we enjoy life more, but for us to really.
Get the best and harness the rewards of this technology. We've got to check those risks early. We've got to detect and mitigate and manage and ensure we're paying attention to the kinds of challenges that this technology can create. And I think for us to really benefit from the promise of this technology, then we've got to understand the pitfalls.
And that's what I see my job as. Allowing leaders and persons who want to engage in this technology to understand the extraordinary potential, but understand the extraordinary risk and find a way for them to be able to maneuver. That rocky risk terrain and be able to then slide, ride that wave with that level of expertise that you see surfers doing, right?
So that's what I think I bring to this space. I just bring a passion and excitement for technology and I just want us to get it right.
[00:09:15] Mahan Tavakoli: I love your optimism that when thought through properly, we can take advantage of the potential that AI provides for a more positive future. Now, one of the things that I understand, Renee, in the conversations that I've had, With different folks in the field of AI is, if anything, data is what drives artificial intelligence.
And I had a great conversation with AJ Agrawal. He has an outstanding book on prediction machines and in essence, Explains how AI to a great extent is a prediction machine. And I married that thought with Gary Bolus, who is head of future of work for Singularity University. Has a great book himself, also had given a prompt to Dolly for an apprentice, and the prompt was a young.
White male, different versions of that prompt came up with the same thing. So what the data was doing, the data is young, white male. Therefore, the prediction of what an apprentice would look like comes out the same way. How can we make sure that these AI systems that are built on the data of the past, don't bring with them the biases of the past and scale them moving into the future?
[00:10:45] Renee Cummings: So I always say that data has a memory. And in that memory stores the great things about humanity and the not so great things about humanity. And once we are using historic data, then we are going to be replicating and repeating some of those challenging painful experiences that we've had.
Data also stores the memory of every great decision that has been. And every not so good decision that has been made, data's memory contains the things that make us absolutely amazing. And the things that we are really not too pleased with, and for us to get that right then we have got to bring a very sophisticated thinking to our dataset and we have got to understand.
If we continue to use historical data sets, then we need to bring a very unique kind of knowledge and understanding of the challenges presented by those data sets. But if we go into this with this kind of bravado as though we're going to just use the data we have, or we're just going to create the systems and the solutions, then we're going in there with the thinking that is not required.
The thinking that is required is that ethical perspective that sets to you, wait a minute, this is historic data. When was this data collected? What was happening at the time this data was collected? What are the kinds of decisions this data has made? Who were the people who analyzed this data? What was the reason for the analysis?
What did that analysis do? Who did that analysis help and who did that analysis hurt? Did we bring due? Into the dynamic when we were using this data, when we were analyzing, when we were collecting, when we were storing, and that's the kind of thinking that you've got to bring. Listen, there's no perfect data set because there is no perfect us.
And there is no perfect society and there is no perfect system. We appreciate that and given those imperfections, it means that we have got to bring a heightened level of due process because what we are doing is building a very sophisticated technology that's repeating and replicating our thinking, and that's our amazing.
Thinking, and that's the thinking that also makes us at times not so amazing. So we've got to bring that understanding. So as a individual involved in data science, that's one of the things that I try to do to bring that understanding to let individuals know that for some communities, data continues to carry a memory of.
And pain and a loss of opportunity and limited access and the inability to acquire generational wealth and the inability to get the kinds of resources that those communities need to thrive. And I continue to let people know that different communities have historically experienced data differently, and those negative experiences are trapped in the memory of those data sets.
So you have got to bring an appreciation to data that says that I understand. That history, I understand that path and if I'm going to help build a future, because I always say to data scientists, you're not just building systems and solutions and products as a collective, you are building our future.
So you have a social responsibility to bring that kind of sophisticated thinking that says that I understand the systemic challenges, I understand the poor decisions that have been made with some of this historic data. I understand that some communities have been marginalized, and then some groups their voice has been taken from them and there's no visibility and they're not represented here.
And what I'm going to do is I'm not here to save the world, but I can certain. Let you know that if I'm going to build a model, a predictive model on this historic dataset, then I'm gonna have some disclaimers there. Then I'm gonna have a conversation about the limitations of this model. And I'm gonna have a conversation that says I understand bias from implicit bias to explicit bias.
I understand the challenges, so I understand the. That's trapped in this data set. And what I'm going to do is use, this technology to really reimagine the ways in which we use data and just reimagine possibilities for all community.
[00:15:33] Mahan Tavakoli: So Renee, I wonder whose responsibility is that?
Is that something that the government needs to advocate for and or enforce? Is it the responsibility of the organization, the startup, c e o, running with their AI company? Or is it a communal responsibility? Who should make sure that those conversations are being had and that level of transparency
[00:15:56] Renee Cummings: exists?
All of the. All of the above. It is important because data is now the lifeblood of our society. I say data has become our D n A, and when we think about the ways in which we are using data, when we think about lineage and data, we are going to have to rethink data in very real ways because that is the power of.
That is the promise of data as well. So we have got to understand at all levels that it is something that is very complex and it is also something that is very complicated data. And for us to get the best out of data, we have got to bring the best kind of. Thinking to deconstructing these datasets, to de-biasing these datasets.
And when we think about bias, of course there are different levels of bias. It could be systemic bias, it could be moral bias, it could be legal bias. There're variations of bias. Sometimes bias is required because bias is a form of discriminating. When we think about data, when we think about statistics and bias and discrimination in statistics are not always.
Negative words, right? They are words that can be seen in a very positive way if you're dealing in the realm of math and statistic and data science. But it also has other implications when we get the negative impact of bias and discrimination. So it really calls for just a new perspective of interpretation that is just deeper than the surface, and an understanding that if you're gonna build something for.
Then you've got to understand the history of that data. Where has that data traveled? Who has that data met? Who has that data? Marginalized, so it's really important. So I think the individual, the data scientists, it's so critical. That's why at U V A, we focus in our school of data science on.
Big data ethics on bringing that ethical perspective of adding the value to technology that is so required to ensure that the data scientists who are trained at the school of data science understand why it is we need to do responsible technology or responsible ai, why we need to build models we can trust.
Why we are committed to data justice. We have a data justice academy that we do in the summertime, exposing undergraduate students to these questions around data. So questions around data justice, questions around data ethics, questions around AI ethics and really designing ethical futures and looking at the relationship between data and society, and those long-term impacts of data on society are so critical.
To us at the University of Virginia that commitment is that we want to ensure that we get technology right? We want to ensure that the interest of the public is included in the ways in which we are designing, developing, deploying, and adopting these technologies. That's why I'm so committed to just sharing my voice and having more conversations.
So from the data scientists to the companies and the companies who are the data scientists that they're hiring, they've gotta think about and of course, go. Because the role of government is to govern, right? So we're looking for good governance, we're looking for oversight. We're trying to ensure that there's compliance and of course there's the right kind of legislation that allows innovation to continue, but to continue ethically.
I always say that ethics and innovation can exist in the same home. It's a perfect marriage if we get it. And I think government has a critical role to play. And I think last year the White House put out that blueprint for the AI Bill of Rights and of course has been working on different policies to really bring the guardrails that are required.
Of course, we have the EU and the EU continues to do very extraordinary work. We have the eu. That everyone's waiting for that to drop, in a kind of way, hoping that the Brussels effect kicks in. And, everybody everywhere in the world starts to do data science and to do ai right?
And to pay attention to those risks. So there are things that we can look at, but I think it's also a responsibility for us the individual. And I try for people to understand that, we don't always have to. We can get involved in the conversation and understand that every day, every time we open our eyes, even when our eyes are closed, we are producing data that's being used, that's being collected, that's being corralled, by the data brokers.
And then of course all of that data is going to one day which is very soon. When I say very soon, the, the same day. Make a decision about us, and that's one of the challenges for me with AI and algorithms. It's that decision making that happen, the agency and the autonomy that we have given to the algorithm.
And we need to know, and we need to be educated on how these algorithms work, how they can benefit us and times where they could easily be weaponized against us.
[00:20:45] Mahan Tavakoli: Renee, kudos, first of all to University of Virginia for spending energy, resources to this effort. And that is something I really appreciate about what you continue to do and what you have done in being a voice for an advocate for this to help more leaders and more people in the community to understand it rather than viewing it as.
Black box that we should trust by default. The more people fully understand the importance of the data, the importance of the algorithm, and have the conversations ahead of time, the more likely we're going to be to have those positive outcomes. Now, , there are some Silicon Valley. Like the Elon Musks of the world that say, the conversation you and I are having is a woke conversation in trying to control AI based on what our values are. Would love to get your thoughts and perspectives on that, on people that say, let it run wild. Don't put your values on the past data sets, for example, with respect to meritocracy.
Whoever has succeeded in the past is most likely succeeded in the future. Don't play around with that data. What would be your thoughts with respect to people that are advocating? Let it flow as it is, rather than try to put your values and systems on the algorithms moving forward.
[00:22:17] Renee Cummings: I think. The entire community around data and AI understand that we've got to bring a responsible approach.
They understand for this technology, particularly AI, to mature, it has got to be a responsible technology. If those values that we are bringing are ethics are emotional intelligence are, mindfulness are an understanding of humanity understanding of intergenerational trauma and how these types of Events have complicated people's lives.
That's okay. Those are the values that we are bringing tech technology because one of the things that we have realized is that this technology in itself is being built by us. And it's being built by many of us who are not bringing the requisite level of emotional intelligence.
And we have seen the crises that continue to be created by this technology. So I think all of these companies understand there are risks. All of them understand there are crises. What companies are trying to do is everyone's trying to rush to market with something. Now we have the. Search engine wars, and everyone is trying to deploy a search engine that's going to just do everything that you've never imagined it could do for you.
But we're seeing challenges. We're seeing lots and lots of challenges it's not about putting our stamp on it, it's, just a really solid group of thinkers and ai ethicists responsible technologists data activists and other social justice. Activists and leaders like yourself and persons involved in government and regulations who understand that, we have done this for a very long time.
When we think about food, do we just put anything on our shelves or people to eat? No. There's an fda when we think about drugs, even movies have ratings now, right? When we think about everything that we have done to ensure that certain protections.
Are provided because we know that children need certain protections when it comes to the internet or when it comes to technology. In particular, when you look at the impact of Instagram. And TikTok on young people, Instagram in particular on young girls and teenage girls, and the kinds of mental illnesses and the kinds of challenges that they now have with their own self-esteem, because everyone is photoshopped, blasted up, and nobody looks.
It's like themselves anymore. And you have young people who are looking at these things, and saying that, they feel I don't belong because, I'm not looking a particular way it just creates this artificial reality that's not who we are.
So when we think about those psychological challenges, when we think about the many children who have seen videos, And they have tried to complete those challenges and have ended up dead. These are real things that are impacting families. The life of a child, a human life. Is more important than anything else.
I've learned that from the criminal justice system. I've worked in homicide, I've worked with people who've been incarcerated, people who have been given life in prison and seen how that kind of intergenerational trauma impacts their generations forever. Children who have parents who are incarcerated, children whose parents have been murdered, these are a real pain that people carry with them.
And now we have technology that. Creating more pain in families. So yes, we know technology can do amazing things, but we also know when we decided to start to develop and design these technologies, we weren't thinking about all of this. When Facebook was developed in a college dorm, it was for people just to get in contact, meet people, have a good time.
They didn't think it would be impacting political decisions worldwide or spreading disinformation. Or just creating the kinds of challenges that we see. So the beauty is that we are creative, we are imaginative, we are innovative. But there needs to be guardrails. There needs to be protection for groups that are vulnerable, groups that are not represented, groups that have been marginalized, groups that need.
An equitable stool to stand on, to get a good footing , to what you know is required of all of us. I would say a lot of people say things in particular because of the internet in particular, because of the speed in which these things spread. So there's a lot of people saying a lot of things, but I think in our hearts, in our heart of hearts, as they say, we know what's.
[00:27:09] Mahan Tavakoli: Renee, I love that. As a father of two teenage girls, I've studied the issue. And I think one of the things that we have been sold over the past dozen plus years is that any attempts to regulate or think through technology will hamper competitiveness. And as you said, whether it's with drugs or food or anything else, we have to have those relevant conversations.
Now, this is a tremendously. Powerful technology that can serve for the good. We have to have those conversations , with artificial intelligence as well. Now, in having those conversations being that you have a background in criminology, you are still involved with it. A lot of law enforcement agencies, Renee, are using artificial intelligence, including.
The Metropolitan Police Department in DC where they are forecasting. Where and when crimes are most likely to occur, and therefore they send more officers and prioritize those high risk areas. Would love to know your thoughts about that and how we can make sure that we are not. Causing more issues, more discrimination in our society as a result of saying, Hey, all we are doing is predicting where the problems in the future will be.
[00:28:50] Renee Cummings: Listen, the police. Across the world have been using predictive analytics for about 20 years now. So they've been using data, they've been compiling an extraordinary amount of data. When we think about the ways in which the police have brought a data-driven approach, which is also known as intelligence-led policing, it takes us back to New York City Comp.
Police Commissioner Bratton, him coming from LA and coming to New York and saying, listen, we're going to use data in the ways in which the subway patrol we're using data in New York City and we're gonna use data to reduce crime. Data and crime is something that they've had a long but turbulent relationship.
What we have seen with predictive analytics is that, We can use data in very powerful ways when it comes to policing. We could use data to reduce police violence in communities and there are individuals who are working in that space. We can use data and AI and virtual reality to train our officers when it comes to reducing implicit bias, which is so critical to the ways in which we see that being acted out on the street and turning in to police violence and turning.
To, gun violence being deployed by the police against citizens. We can use data science in very creative ways to design early warning systems for us to know who are the troubled officers, who are the officers who continue to get into violence situations. And save these officers from themselves and get them the kind of treatment that they could use.
And then we could use predictive analytics in the ways in which we've been using it, which is we continue to over police communities that are already overpoliced. And this is why in my work at the University of Virginia and in the process of designing a public interest tool called the Digital Force Index, and what we have been doing is that we have been.
All the surveillance technologies across the US and we're coming up with different scores for different communities depending on how much surveillance technology is in your community. And this is a way for law enforcement to see whether or not the kinds of technologies that we are deploying in communities are having any real impact when it comes to reducing crime, violence, and.
Or is it that we are just getting ourselves involved in these vanity projects where we believe the technology is the answer to anything that is crime and criminality. And what we're seeing is that technology and the deployment of an extraordinary amount of technology in a community does not reduce crime.
What reduces crime is paying attention to the challenges, bringing things into the community that could lift these community up. Focusing on mental health, focusing on socio. Economic conditions, what we consider would be the criminogenic social conditions that may move an individual into a life of crime.
So when it comes to the police using these technologies, we've got to be critical as well, because there's a lot of pseudoscience out there, a lot of pseudoscience, and people believing that they can design an algorithm to say who's criminal, who's not criminal, who walks like a criminal, who doesn't walk like a criminal, and who's going to perpetrate crimes.
We have seen the challenges with facial recognition. We have seen how this technology created the wrongful arrest of black and brown men, or the ways in which it's unable to really work with persons who are black and brown, people who identify as female and the many challenges of this technology and these challenges from a policing perspective.
Are just not challenges. They become civil rights challenges. They become human rights challenges. So when it comes to the deployment of algorithms by police officers, there are positive things that can be done, but it needs to be done ethically, not in a serendipitous or capricious manner. We need to ensure that law enforcement agencies understand the ethics.
Around the deployment of algorithms or using an algorithm as an undercover cop or using an algorithm as a detective or using facial recognition. And we need for officers to know that when we are thinking a about data, And we're thinking about harnessing the best from data. We've really got to understand the challenges of these communities because what we have seen is the technology has been deployed in law enforcement communities forever.
But crime is not going down in a lot of these communities. Homicide keeps increasing. So you've got to ask yourself, we have all this technology out here, but it's not delivering the kinds of results. So what's missing? What's missing is that interaction. What's missing sometimes is that old school policing where you get to know the people in your community and there is no technology that could replace that human interaction and that kind of mentorship, and the ways in which officers would know communities in the past and really have the kinds of conversations that are necessary.
So I'm all for big. But I'm for ethical big data and I'm for officers using more of their imagination and using more of the techniques around community policing and understanding what requires public trust and how do we build public confidence and the things that seal police legitimacy. And just not becoming lazy and thinking there's an algorithm that's gonna turn into a silver bullet and just solve everything for you because that is not going to happen.
And as well as officers understanding the dangers of trying to use algorithms in ways in which they should not be used. And one of the other things that we see when it comes to the police and data and technologies that many. There's a lot of hush and a lot of secrecy around the budgets that are being given to these third parties to develop these technologies.
And once there is secrecy and once there's that veil that covers the procurement practices that we know that these agencies are not doing what they should do. And they may be looking for a quick fix, but it's not going to happen. Technology still needs a human intervention, a human in the loop, and sometimes not a human in the loop, but a human creating the loop.
Human moving the loop and a human just there because mean at the end of the day, technology is not going to be the answer to every problem. We're never going to be that committed to techno solutionism because so many times a technological response is not required. Many times a human touch, a human idea, a human smile, a hug these are the things that change the.
[00:35:34] Mahan Tavakoli: And that's why it's important for us to understand the potential of the technology to serve as a tool. Renee, a couple of months back, an African American man. Was determined based on facial recognition to have been the person that had potentially been a suspect for a crime.
[00:35:55] Renee Cummings: Is that the case of Robert Williams in Detroit, where they said that he was in a mall stealing watches, and he was arrested in front of his children. It's the classic case of the a c l. Focuses on that. Looks at misrepresentation and wrongful arrest
[00:36:09] Mahan Tavakoli: The officers didn't question the technology and part of the point that you make is that the technology can serve as a tool. In many instances, it's been outsourced to technology rather than that humanity, that human engagement, which is a critical part of it, whether in policing or elsewhere the other.
You mentioned, I wanted to highlight, you said there is a certain opaqueness to some of where the resources are allocated and how it's done. For example, nothing against Peter. Thiel and Palantir, which is one of the top providers of artificial intelligence services to US government, more than quarter billion dollars.
Peter Thiel has a very specific ideology himself and he drives it. So I would imagine a lot of people that are using. Palant here. A lot of government agencies and organizations aren't clear on how the decisions are reached, what the algorithm is but still it's something that they rely on. So we have to question it at all levels, and that's the transparency that you talk about that is really important before we implement these.
[00:37:28] Renee Cummings: Definitely. So it's always about accountability and transparency and explainability, accountability, auditability. These things are so critical, so many things for us to just deconstruct here. When we think about the arrest of Robert Williams by facial recognition, we're not thinking about the extraordinary trauma, his children and his wife.
Experience by him being arrested at home, and that is a trauma that you cannot take away. So when I speak about really understanding data and why we need to bring a trauma informed perspective to the ways in which we're deploying technology is because these technologies are replicating further trauma.
Not only that, he was arrested for 15 plus hours. He had to. His job and say, I was not coming in, or his wife had to call his job and say Robert was arrested. We have to think about all those factors that create. Further challenges for individuals. And then when we are thinking about these vendors, think of that case that continues to just like rare, it's ugly.
Yet in Allegheny County when we are talking about that algorithm that is being used in child welfare and child protection and a recent case of. Parents, and I think the parents actually identify as disabled. They have some psychological and neurological challenges and they brought their daughter to the hospital because she was dehydrated and they were told by the pediatrician to take her to the hospital because the child was not eating.
And of course while they were in the hospital they realized that they got a visit from child protection. And all of a sudden their child was taken away from them and they now had to have supervised visits with their own child because disability has now become a data point for irresponsibility.
When it comes to a parent, there are many parents that have disabilities. There are parents who were visually impaired, parents who are hearing impaired parents who have neurological diverse situations. It doesn't mean they're bad. It doesn't mean they cannot parent it. So when we're thinking about the kinds of data that we're using to create these risk assessment tools, these algorithmic risk assessment systems and the kinds of challenges they're creating for families now.
Yeah. An algorithm said that these parents, are no longer worthy of taking care of that child. But are they thinking about the experiences for that child? Are they thinking about the fact that disability does not mean that you are not able, it doesn't mean that you're not able. And those are the things that we've got to think about.
And when we think about the ways in which. Persons who have to use the technology may not understand the ethics because many of the social workers who were dealing with the case said, we've been using these algorithms. We've been trained to use the system, but we don't really know.
What's in this black box? We don't really know what has been used to create those data points because it always comes back to intellectual property, proprietary rights whether or not we have access to the black box. Although we've had one or two cases over the last few months where individuals have.
Won the right to now challenge the vendors and the developers and to really get inside that black box and see why a particular decision was made. The other issue is that many of the designers and developers of those opaque technologies still don't understand exactly why. The algorithm is doing what it's doing, but if an algorithm denies someone bail, if an algorithm denies someone parole, if an algorithm says that you need to be sentenced for a particular time, it means an algorithm has an extraordinary amount of power.
I'm committed to collective. Augmented intelligence. I'm committed to the human brain and that machine, brain working together to give us the best decisions in real time to inspire creativity and innovation and really to spark the kind of thinking where we could just reimagine this world in real time.
That's what I'm committed to. I'm certainly not committed to an algorithm having an extreme amount of autonomy and making decisions that I cannot. Investigate or decisions where there are no redress we've got to really rethink some of these things and understand that people are involved and lives are involved and children are involved, and families and communities and futures are involved.
And you can't play with someone's future because if you play with someone's future, you are playing with the future of a generat. And That'ss, why we need those guard.
[00:42:14] Mahan Tavakoli: Renee that also goes along with what Louie Rosenberg, who is he's c e o right now of unanimous AI was a pioneer in augmented reality, virtual reality.
Part of what he says. In that his fear is people driving for AI driven decisions as opposed to augmentation of decisions. And in many instances, as you mentioned, a lot of times even the companies themselves and the developers don't fully understand the. Decision making process that the algorithm is going through.
So they can't point, there are so many different data points that they can't point to. This is the exact reason why, which is why that involvement in humanity is really important. So my question is for you for. The business leaders for community leaders, for governmental leaders, how can we influence the direction that this AI goes in?
I love the fact that you have the optimism about the potential of the technology, which is outstanding, and you warn us that we need to have guardrails around it. So what can we. As leaders of organizations, nonprofits and government agencies to make sure that we guide the algorithms, the conversation that the outcomes to a positive future rather than a dystopian one driven by the algorithms themselves.
[00:43:55] Renee Cummings: I think what we need to understand is that this technology needs. This technology needs all of us. This technology cannot do the great things that it is ready to do without our involvement. We need to understand that if we design it ethically, it means that we are designing it in a way in which it is going to really help.
Instead of harm us, it means that if we are bringing diversity equity and inclusion and bringing a trauma informed and justice oriented perspective, it means we are stretching the imagination of this technology in ways in which it is yet to be stretched, and we've got to understand for us to profit.
From the potential the promise and the power of this technology. We have got to understand the pitfalls and the fact that this technology can create extreme prejudice and it can lead to the persecution and the further persecution of certain groups. And if we are to get this technology right, and if we are to do AI in a way in which.
Equitable, and it is inclusive, and it is diverse, and it is justice oriented, and it is trauma informed. It means that we have done an extraordinary job as being a citizen of this world, and when we leave this world, the generations that come behind us are going to be super proud.
[00:45:32] Mahan Tavakoli: The generations coming behind us will be super proud of us as the podcast has listeners in a hundred plus countries, including Trinidad and Tobago, and I know all of the listeners, especially the ones in Trinidad and Tobago, are jumping up and down.
Are proud of you, Renee. I love, the way you communicate this, Renee, with tremendous positivity for the potential of it. Making sure we are aware of the need for those guardrails as well, which is why I appreciate the fact that you take the time to communicate to the outside world.
So for people who are not immersed. Deep data and in artificial intelligence, however, they listen to you and they.
I get it. I want to understand more, to be able to have a positive impact. Are there resources that you typically recommend for them to read, to watch things to do, to understand it enough to ask the right questions? Because one of the things I'm hoping to do in these conversations, Renee, is inform the leaders.
That listen to the episodes enough so at least they know what questions to ask. We might not have the answers, but at least you know what questions to ask. So are there any resources you find yourself recommending
[00:47:00] Renee Cummings: for that? Definitely. I think if you wanna watch something on Netflix, there's coded bias and it really features many of the women who have made us really rethink the direction that we have been traveling and the speed at which we have been traveling.
It gives you a really great insight to technology and the kinds of harms that could be created. If you're looking for something to read, there are several books that you need to read. Weapons of Math Destruction by Kathy O'Neill is definitely one race after technology by RHA Benjamin, and Viral Justice by RHA Benjamin, you need to read artificial unintelligence and.
More than a glitch by Meredith Brard is critical. Of course. Sophia Noble's algorithms of oppression are very critical. And of course, there's an extraordinary ray of books that I have here. I can read out all of them, but I think if you get on those you would understand what needs to be done and what your role is and why we cannot take this lightly.
And I always say I was not. For the invention of the printing press and the steam engine, but I am certainly here for the invention of ai, and I'm living in the age of ai and I am going to be a part of that conversation because I'm in love. With ai, I'm in love with data science. I'm just in love with the things that we can do with this technology.
We can correct so many things that we did incorrectly. We can just change the direction of so many groups that didn't have the kinds of opportunities that so many of us have had. We can do so much for children. When we think about the ways we could use virtual reality to reduce child abuse, to reduce child hunger, to really protect children and to give children that possibility where they can just really dream of being anything, and then having technology as a tool, as a companion.
As an aid when I think of children who are in our neurodiverse population and how this technology can work for children who are born visually impaired and adhering impaired. And what about data science that says it can make us young and beautiful forever. That's the one that I really like.
So what do you think about the great things we can do? The ways in which we can connect, the ways in which we can enjoy entertainment. Now we can probably step into the movie and be a part of the movie. I look forward for all of those great things, but then I also look forward to the fact that we're doing this thing right and we're ensuring that there is equity.
We're closing the digital divide because we don't want this technology to widen the digital divide, and we just want to give people an opportunity to. Their best lives and thrive. And if we do that, then we've done the work that we need to do while we are here on this earth.
[00:49:51] Mahan Tavakoli: So beautifully put, Renee, I am in love with your leadership in this space and you championing and helping all of us contribute to that better future.
Thank you so much for this conversation, Renee.
[00:50:09] Renee Cummings: Thank you so much for inviting me. It's been an absolute pleasure. Thank you again.