269 The AI-Powered Enterprise: How to Make Your Business Smarter, Faster, and More Profitable with Seth Earley, CEO Earley Information Science | Partnering Leadership AI Global Thought Leader
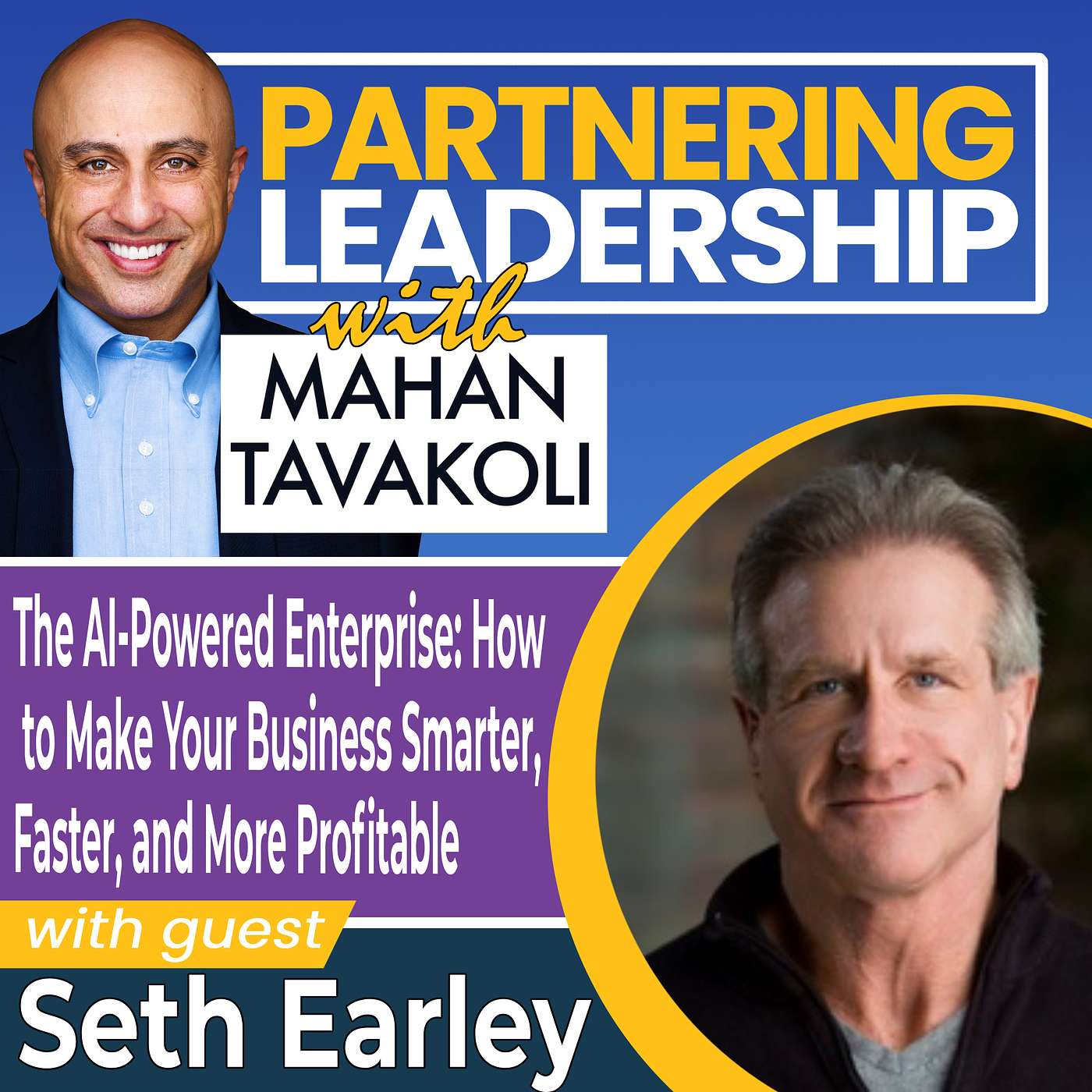
In this Partnering Leadership conversation, Mahan Tavakoli speaks with Seth Early, CEO & Founder at Earley Information Science and Award Winning Author of The AI-Powered Enterprise. In the discussion, Seth Earley, an expert in knowledge strategy and artificial intelligence, emphasizes the crucial role of understanding processes and use cases in successfully integrating AI tools within organizations. Seth Earley goes on to highlight why there is a need for organizations to build libraries of use cases and stresses that AI alone cannot solve complex business problems. Then Seth Earley shares why, despite AI advancements, organizing and sharing information effectively through information architecture is still essential, increasing the significance of data models and accurate data for optimal algorithm performance. Finally, Seth Earley discusses the potential dangers of generative AI, such as the proliferation of misinformation and deep fakes. However, he also highlights the productivity-enhancing potential of generative models when used in conjunction with human expertise.
Some Highlights:
- Discover the power of understanding processes and use cases in implementing AI tools. Learn how building libraries of use cases can impact and influence organizational processes.
- Uncover the crucial role of information architecture (IA) in the age of AI. Despite advances in AI, IA is still necessary for organizing and sharing information effectively.
- Understand the importance of having good enough data for AI applications. Learn how to evaluate data and determine what is valuable for solving problems and providing insights.
- Explore the impact of generative AI on humans and society. Discover the potential dangers of misinformation, deep fakes, and the productivity benefits of automating specific tasks.
- Understand the role of automation and human expertise in copywriting and AI. When using automated tools, discover the importance of asking the right questions and providing enough detail.
- Learn why AI is not the ultimate solution for knowledge management but rather a tool in the toolkit to enhance human effectiveness.
- Discover the significance of knowledge graphs and ontologies in organizing and managing organizational data. Learn how they can improve data quality, ownership, and usage.
- Learn the importance of understanding the customer journey and digital body language to make informed decisions. Discover how organizing information through ontologies and knowledge graphs can create seamless experiences.
- Explore the potential of AI in transforming organizations and the need to measure outcomes and communicate insights effectively.
- Discover the potential consequences of not involving the business side early enough in AI initiatives. Learn the importance of a real business case and relevant data collection for successful AI projects.
Connect with Seth Earley
Connect with Mahan Tavakoli:
***DISCLAIMER: Please note that the following AI-generated transcript may not be 100% accurate and could contain misspellings or errors.***
[00:00:00] Mahan Tavakoli: Seth Early.
Welcome to Partnering Leadership. I am thrilled to have you in this conversation with me.
[00:00:05] Seth Earley: Great to be here. Thank you for inviting me.
[00:00:08] Mahan Tavakoli: Seth, I've learned so much from you so can't wait to get some of your insights to share with the audience.
But would love to first start out with whereabouts you grew up and how your upbringing has impacted who you've become. Seth.
[00:00:22] Seth Earley: That's a great question. I grew up in New York and until I was a teenager, then I moved to. Boston. My father was actually a scientist. He was a researcher. He worked at bell Labs at the time.
It was G t E Labs. Prior to that was Bell Labs. And he was the inventor of the Helium Neon Laser which was one of the first lasers in existence. And that led to all of the laser stuff we have today. He he was a brilliant man.
He was not formerly educated himself but he also worked on the Manhattan Project and World War ii, and I did not recall that. Used to talk about Robert Oppenheimer, used to say, Oppenheimer this and Oppenheimer that when I was a child. And of course, I didn't have any context. And it wasn't until I was an adult and I read American Prometheus, which was the story of Robert Oppenheimer.
That I remembered all that. And so he was actually recruited he was working in an explosives laboratory in Australia. He was stationed in Australia, and he was recruited to come work in Los Alamos New Mexico. And of course the conventional atomic bond, original fission bomb is set off by conventional explosives.
And that was his area of specialty. So I don't know exactly what he did, but I know he worked directly with Oppenheimer. So his influence was really to engender a a love of science. When I was a kid, he used to bring me into the labs. He used to show me the experiments.
Back then they were actually physical experiments that they'd be running. He'd show me how to melt things with induction electrical currents, and then show me what the early lasers could do. Would walk me through the labs and then he would bring home surplus equipment that was just out for disposal.
I tear that stuff apart radar equipment and I'd salvage electronics parts and I'd build things and I built, radios and built, amplifiers and all of that. And then I actually got bored with electronics. But I still love science and chemistry and biology.
So my undergraduate was in chemistry, started in biology, but I ended up getting a degree in c. And that was pure chemistry as opposed to chemical engineering. And then for graduate school, I ended up going to the dark side. I went for business administration.
And I like to say I have an almost MBA because I did two years full-time study, took an incomplete in one course, and I remember the instructor saying nobody who's ever taken an incomplete has ever finished this course. Then when I went back to finish a couple years later, they changed the accreditation wanted me to start again.
But it's funny because I'm frequently addressed as doctor early, all these publications are saying, doctor Early and Chat g p t told me I had a PhD and that I wrote all these other books, which kind of brings us to generative ai, which is really one of those interesting things in terms of our inflection point around ai.
The chat g
[00:03:04] Mahan Tavakoli: PT said, you are a doctor.
[00:03:07] Seth Earley: We won't question that, Seth. No, we have to question I don't have an MBA and , I'm not a PhD so I can't say I do, even if the interweb says I do, even if chat C p t does, but that's one of the flaws with generative AI is that, it sounds plausible.
And that's the whole idea of the beauty of these language models is they sound plausible. But they can be completely factually incorrect. . I recently wrote an article about that, and I asked who Seth Early was, and it didn't know who I was until I asked who Early Information Science was.
Then it said it was founded by Seth, which is really interesting. Then I asked who Seth Early was, and then it, at one point it gave me all these book credits that I taught graduate school at University of Pennsylvania. It said, I, did all this stuff and it was like, thank you Chuck pt. But no it sounds plausible, . Seth,
[00:03:56] Mahan Tavakoli: That does raise a lot of questions around the potential use and misuse of these generative models. First, I love your father's story, . In that you said he didn't have much of a formal education, but it was his passion that got him involved in science. How outstanding to see that, and for you, you don't need to be a PhD doctor to be someone who is at the cutting edge of science and artificial intelligence.
The degrees you have are good enough. You have played around, you understand them well, which is incredible. Now, you mentioned that a generative ai, and I wonder what impact do you think that is going to have on us as humans in that we tend to believe what we see? With video, I've seen fake video that is as good and you can't really distinguish right, if the person actually said those things or not.
And with generative ai, as you mentioned with real conviction, you read sentences that are giving you incorrect information. So what impact do you think that is going to have on our thinking and us moving forward?
[00:05:15] Seth Earley: There's a lot of dangers, obviously, when you start thinking about proliferation of misinformation, and, that's already happening with conventional programs and conventional technology.
And then, I think that generative ai, which can, create these deep fakes,
So I think that's going to be. Very problematic in society. That's what's one of the challenging things, however, on the upside in terms of productivity, people are afraid it's gonna replace jobs, replace humans, they'll replace parts of jobs,
and it's not good enough to replace all of jobs. And, more and more of these things are going to get automated, entry level job positions where say, call centers are being increasingly automated. Copywriting can be increasingly automated, but you still need human expertise.
You still need human judgment. You still need more knowledge than what you're asking of the system, and it's about asking the right questions to generate the right responses. I just did a workshop for a charter school of the Phoenix Academy. I'll plug them here.
They serve underrepresented populations up here, Massachusetts. It's a great program and I'd love to see it scaled, nationwide. And one of the things we talked about is what is the impact of Chachi d t. Not only administrative work, but also in the classroom. You didn't touch a lot in the classroom but I said, , to teach young people how to effectively use these tools is to not say you're going to use this, to plagiarize or to do your work, but you need to think critically.
You need to understand what you're asking for, and you need to give it enough detail and enough nuance to ask the right questions. So I showed examples of, write a job description versus write a job description for a fundraiser and a development director working for a charter school serving this population, et cetera, et cetera, versus write a press release versus write a press release.
The opening of a new science and technology center for the Phoenix Charter Academy serving this population and why this is so important. STEM is so important because underrepresented populations have even fewer opportunities, right? So we need to make sure that we use these tools to level the playing field as much as possible.
So the whole idea is teaching children how to use these tools, teaching people how to use these tools, teaching people how to use them in their jobs.
You want to do research, you wanna do a webinar, you want to write a white paper, don't take it for what it is, but use it to generate ideas. Use it to put together an outline, use it to ask very specific research questions. That's the way to use the tool. But then when you start thinking of the larger enterprises and how they need to prepare for building artificial intelligence and machine learning applications, They still need the data, they still need their own information and they still need to fix the data problems.
I even asked chatt, PT said, is chatt PT going to solve master data problems? It's no, . It's not. Period. And is it gonna be the solution to knowledge management? No, it's not. It's a tool in the toolkit, and that's how we have to look at all of ai. These are tools to make humans more effective,
it's to help people do their jobs just like any technology over time, anytime we've seen automation, steam shovels at the turn of the 20th century, put all those ditch diggers out of business,
but wait a minute, people didn't like digging ditches, and guess what? Now you can build skyscrapers and highways and do all these other things What we're doing is we're going to cause massive disruption, in the labor force, in people's jobs, in work. But what we need to do is we need to anticipate that, and we need to mitigate as much as possible.
And I think the tools that are leading to these problems are also going to be part of the solution, being able to make this stuff accessible to people, make it less mysterious, help people understand how they have to configure this and really open up their eyes to the fact that you don't need a PhD in data science and computer engineering you don't, in order to understand business problems and to start applying these tools to business problems,
[00:09:17] Mahan Tavakoli: That's outstanding, Seth. On the organizational side of it, one of the interesting things that I saw, Chad, g p t do is at least with many of the CEOs and organizations I interact with for years, if not decades, there have been aspects of artificial intelligence used in their organizations.
So many of them use applicant tracking systems that have an AI behind them. However, it raised the awareness of a lot of CEOs and senior teams. To, whoa. Our business model could all of a sudden be disrupted. Yes. And we better come up with a strategy on artificial intelligence. So that's why I also read your book, the AI Powered Enterprise, the Power of Ontologies to make your business smarter, faster, and more profitable.
In this context Sure. What is an ontology? Before we talk about how business leaders and CEOs can think about this with their organizations. Sure.
[00:10:26] Seth Earley: Think of an ontology as a framework for knowledge and information. It's a structure, it's a scaffolding and it's made up of things called taxonomies. Taxonomy is simply a list of values. Parent, child, whole part like. Boston is part of Massachusetts, which is part of the us that's a hierarchy. We usually see hierarchies of product information and product categories.
We have different document types and content types. We have different types of customers. We have employee roles and positions, we have processes, we have all sorts of artifacts and sources of information, in various domains of information, and we have to organize that. We have to have standard ways of looking at things and naming things so that we can share them.
So we can find them, and a lot of people think, oh wow, with all the advances in ai you're not gonna need that anymore. But the fact is and I wrote an article on this several years ago, you can't have AI without the information architecture. I wrote an article called There's no AI without ia,
there's no artificial intelligence without information. Architecture is at the core, at the foundation. There are data models, and even if the data model is created by the vendor or within the algorithm, it's still there. And the more accurate your data is, and the more precise your data, the more complete your data, the better your algorithm will operate.
People think that AI will take bad data and make it good. It doesn't work that way. There can be some tools that will help with data quality, data cleansing but you really do need reference data. And so when you start thinking about this whole idea of taxonomies, there's lots of taxonomies in an organization.
So we're working with a life sciences company and there are diseases and drugs and indications and mechanisms of action drug targets and biochemical pathways and molecular entities and all of those things. And those are all certain vocabularies. But then you have to say what's the relationship between them?
What's the indication for this disease? What is the treatment? For this indication, what is the biochemical pathway for this mechanism of action? What is this target based on this particular mechanism of action? So all of these things start interrelating. You can start looking at it from the perspective of what are my products and what are my solutions?
What are the products that go with that solution, what are my services that go with those solutions? So you start building these relationships and when you have all these taxonomies that describe a domain of knowledge, so I mentioned life sciences as those things of insurance company would have risks and regions and products and services and content types and employee types and customer types and business types and all of that.
And then when you say, here are the risks in this region, here are the products for this type of business, here are the risks for this type of business. Those relationships become A scaffolding, become a knowledge architecture you can think of. If you ever go to I M D B and you see all the movies and you see the directors, and then you can go from what other movies did this director direct, and then what actors were in that movie?
That's an ontology, so an ontology is a framework for information, facebook uses an ontology, friend of a friend and what schools did you go to? So when you build this for the enterprise, you're building a knowledge network. You're building a knowledge scaffolding. These are called knowledge graphs.
So the ontology is that framework, it's all those different sets of organizing principles. There's different taxonomies of your products and services and people and customers and all that stuff. You have the relationships between them. You're building the ontology. When you then use that to access the information and the data, you have a knowledge graph.
And the knowledge graph allows you to. Navigate, , to discover all those different relationships, to find out what data you have to understand the quality of that data to understand who owns that data, what it's used for, you can start cataloging that data. You can start exploiting and leveraging that data.
You can start using it in different ways. And then when you have new information and new knowledge, you have a place to hang it, you have a place to put it in that scaffolding. So this is what's so important to organizations. They have to get their knowledge house in order. They have to get their data house in order.
And, their four domains that we look at, really it's product, it's content it's the customer and it's knowledge, and knowledge and content can have some gray areas, but then of course there's financial data that wraps around all of this. There's metrics that wrap around all of this, but really what you're trying to do is orchestrate the experience of a customer or an employee.
You're trying to personalize a scale, and you do that by orchestrating the information that they can access through their journey, when an employee has certain tasks, they need certain information sources. Where is that information? How do they access it? How do they filter? How do you surface it for them in anticipation of their task?
So that's why journeys are so important. That's why understanding processes are so important. What you do, is you build libraries of use cases for the organization, both internally and externally. And then those use cases can be used to describe job positions. Those can also be used to describe processes that we want to impact, that we want to influence with a particular tool.
We're looking for an intervention throughout a process. So if we understand that process, then we understand where we can have an intervention, that's where we can insert use of an AI tool. AI does not solve the whole business problem by itself, maybe there are more things that are self-contained but as you said, it's behind the scenes a lot,
word processing was actually one of the first applications of ai. Back in the day, before you had word processing, before you had Word, you had to hire type setter. You had to hire graphic designer. You had to hire somebody who knew all the control keys and all of that. And you had to, bold and this, and it was just really complicated.
They took all of that out with Word. We don't say we're gonna open up our AI and write a document, we're gonna open up Word. So it used to be the saying was that no AI worked. Anything that worked was no longer called AI back in the day, 10 years ago. Because as soon as it worked, they called it something else.
Spout check, but now everything is ai, everybody says everything is ai, AI powered. Oh, we have machine learning. Oh, we have magic algorithms. But really at the end of the day, their algorithms that take an input and produce an output. And what you need to understand is what is that input and what is that output?
And what is that process that you're trying to enable? And if you don't understand that process, you can't automate it, you can't automate what you don't understand, and you can't automate a mess. So the first things you need to do is understand the process. So mapping your processes is critical.
That's the starting point. And then looking at the data that's required at each step of that process, customer going through a journey, they're going from. Trying some software which is free to going through and then paying for features that's a journey. What information do they need to make that decision?
What's gonna catalyze that next stage of their journey as they go through that phone, what's gonna cause them to actually make that decision to buy? And that is done with digital body language, that's the stuff that people do every time they interact with your organization.
So understanding that journey and understanding that digital body language and then reacting to that, giving them the stuff they need that aligns with their mental model, aligns with how they think about their problem, how they think about solving their. And that's where the ontology comes in. That's where the knowledge graph comes in cuz because it's organizing all of that stuff so that you can orchestrate that experience.
And it works for employees too, employees don't want to come in and have, disconnected processes and their job is frustrating cause they can't find stuff and they can't get to the answers.
When you think about a customer journey, it's a knowledge journey. What do they need? They're always asking questions and seeking answers. And your employees need to get that stuff. So again, when you start looking at this holistically, you can see where you can apply machine learning. You can see where you can apply to a part of a process,
if some vendor comes and they say, oh yeah, our algorithm will do it all. You don't have to think of anything. Yes it's a black box and it's gonna give you these results. We'll just. If they say, oh, it's proprietary, we can't tell you, go somewhere else,
they should be able to explain the inputs, explain what the system does, and explain the outputs, and it really is about process efficiency and effectiveness. It's really about building capabilities and really knowing, where you are in that learning curve. Because there are going to be certain things that you want to try to do, but you need to have the foundation place to do.
[00:19:19] Mahan Tavakoli: Seth, a couple of questions about that. First of all, in looking at the overarching ontology and information structure for the organization when you're working with organizations of different sizes, what group or who takes that responsibility on? And then secondarily, in many instances when I have conversations, as you mentioned, the quality of the data is important.
That said, the quality of the data can always be improved. At what point is the data good enough? For the application of
[00:19:53] video1319027977: ai.
[00:19:53] Seth Earley: So I can translate it this way. Make the punishment fit the crime. You don't wanna make people go through hoops when there's no sense to it.
If you think about moving, your house, you box stuff up in your attic. You don't need to organize your attic. If you're never gonna go up there. And at the same time, if you're moving into a new place and you say you'll clean your attic later, you're never gonna clean your attic, right?
So when you're going to new systems and processes, that's the time to look at your data, and that's the time to look at the foundation and kind of the benchmarks. What's good enough. What's good enough is when you can solve the problem of the process, and when you ask the question of what can you do, if you had this answer, if you had this answer or you had this information, you had it faster, or if it's more accurate, and then go backwards and say, what do I need to produce that answer?
What do I need to solve that problem? What do I need to build that capability? So it's all use case driven. I mentioned use cases before, right? Journeys break everything down into a journey. Customer journey, employee journey, whether it's a set of processes. It could be about software development, DevOps, it could be about.
Product development. It could be about customer service, it could be about, new offerings, any of those things. What's the information People need each step of that process, and when you start to look at that and you say, oh, we can't answer that question. Why? Ooh, we don't have the data.
Oh, why? We didn't capture it over here. Okay, why? Let's come up with a mechanism so we can start capturing that. Now let's build a process around that. Now let's build compliance mechanism to make sure we're doing it correctly. Now let's build metrics so we can measure that we're doing it correctly.
We have the right level of data quality to support the process. And if it's not supporting a process, if you can't build a use case or identify a use case, don't bother. It has to have a value when people talk. Oh, we're building a knowledge graph. I talked to one company and they invested half a million dollars in the knowledge graph, and they said, that's great.
Wow. Wonderful. What was the business case? And they said, oh, we didn't have a business case. Really? You didn't have a business case? We just had to get started. Okay. You can get the funding and have a science experiment. You not have a business case. God love you. But most people have to have a business case.
Now you can have experiments, you can, have learnings. You can have things you're gonna throw away. But for the most part, you want to have a business case, what's the problem you're trying to solve? What's the business value? And then back into that is the data there to make those decisions.
We don't wanna clean up data for the seek cleaning up data that's like going to your attic and organizing newspapers that are sitting up there into, categories why, you don't need that. So we don't want to go into data sources that we don't need. Maybe you want to discover what's in those data sources.
They call 'em dark data. For the most part, a lot of that stuff doesn't have a huge amount of value anyway. Maybe there's some archival value, maybe there is some stuff you can mine from it, but there are techniques for doing that. But it really needs to focus on a process that you can measure, and it's about measuring base models.
So everything should have a baseline and everything should be measurable, and you can measure that across the entire customer journey or entire brain journey. Now, what are the problems with that? It's easier said than done because the data is disparity, the data is disconnected, it's owned by different groups, it's sitting in different applications.
So what I'm saying is by no means trivial, but it's critical to do this. When you start looking at the big picture, what you're trying to do is you're trying to speed up the information metabolism of the organization. Meaning you're trying to get information in people's hands so they can make better decisions faster,
meaning getting to market faster. Reacting to competitive threats, faster, reacting to customer needs, product differentiation, all of the things that are happening in the marketplace. If it takes you, six months to get a product on your website, that's a problem. It should take six hours.
There are companies that literally take weeks and months to do that stuff because the processes are so disconnected. So it's looking at where you can speed up those processes and speed up those information flows and improve the quality of those decisions. And when you can do that, and you can justify and when you measure it, you can also justify it,
and then you can see what the impact of your.
[00:24:16] Mahan Tavakoli: And that's why I love your insight, Seth. You beautifully answered a concern that a CEO I was having conversation with last week had, he was confiding in me and said, you know what? We've wasted so much money on different field tech initiatives. And I would say there are two contributing factors in my view.
One is that the people who are more on the business operational sides didn't get engaged early enough. So they relied on the people who understand the technology to attempt to come up with their experiments and to your point, It wasn't a real business case that was made ahead of time. So the point you're making can add real value to where can we add value to the organization through the use of ai with the data that we have. And if we don't have the data, how can we collect the right data in order to meet those business measures So this doesn't become another wasted tech
[00:25:25] Seth Earley: initiative.
That's right. And I think you hit the nail on the head. If the business is not engaged, and sometimes the business is engaged because they don't understand, they don't understand the capabilities. Sometimes executives will nod their heads and say, yeah, I understand. Because they're embarrassed.
They think they should understand, and many times they don't. So one of the things that's important to do is engage people in such a way. Where you're clarifying things and you're, explaining things in a way that does not impact people's egos, you don't want them to feel like they're dumb.
We did a project for a company that made little connectory do dads and it was a 13 billion company. So you had, these are all like a 10th of a cent each. They had to make a lot of these things and they had a huge range of applications and, they had a motorcycle racing connector division.
That's how nuanced and fine grained they were. And they were doing a digital transformation. It was about 25 million. And the c o said, This is a drop in the bucket. This is a rounding error. I know I shouldn't say that with vendors in the room, but it is, and it was, our piece of it was two, 3 million.
But if we hadn't done the data, that project would've failed. That project would've failed. Period. Full stop. It would not have been successful because we had that foundation, that 25 million project, that led to a multi-billion dollar increase in the market capitalization of that. So now when you talk about those kinds of numbers, fixing the data is trivial. It's trivial, it is a rounding number. A rounding error. And the companies that are doing it with acts of hers and acts of hers don't scale and they're not sustainable, and you burn people out and your competitors are automating while you are doing acts of hers and manual efforts, that guess what is not gonna last?
You may have the volume and the size and the, market momentum and the market presence to do that for a while. But you will be disrupted. You will be disrupted by people with better data. We are then making use of these algorithms. The data is more important than the algorithm.
[00:27:32] Mahan Tavakoli: I love the way you put it, Seth and I modify something someone else had said with respect to ai, which was go in the organization where the data is. I love the modification and the point that you add, it's not just where the data is, where the data is, or you can gather the right data, but where it is an friction point or an opportunity that's right for real business insights to have an impact.
That's why the investment then is worth it because it's addressing a business challenge. It is not implementation of AI because that's where we've got the data, right?
[00:28:14] Seth Earley: It's an information leverage point, right? It's reducing the friction, but it's also leveraging that information, meaning it's gonna have a downstream impact that is disproportionate to that particular friction point.
So when you release that friction and you are leveraging that data and unleashing that data for many downstream systems and applications. So it really can be, exponential in terms of the capability, in terms of the efficiency and the effectiveness, because that data has lots of purposes and lots of usages.
I have this little sculpture that kind of informed my logo, have it behind me, and I'll try not to drop it, but it's this cube, right? And when you look at this cube has a lot of structure to it. I look at that as the ontology, right? That's the ontology.
There's structure as you rotate it, there's infinite ways of looking at that information, that is the application of your data. The data has to have that structure, which is that inner crystal and structure that you see with those different pieces. And then as you rotate this, you see all the different angles on this.
It's infinite. The applications are endless once you have that structure. But you have to have that structure because again, AI is not gonna run by itself. It's not gonna run on bad data. It's not gonna run when you don't understand what you have, and when you don't have a handle on it. When you can't describe, the things you need to understand is this, and about this.
What is this stuff? And tell me what I can understand about it by the thousand contracts in a pile, how do we tell 'em apart? It is a contract. It is about, labor or a statement of work, or a transaction or a purchase or whatever it is. But that's what you need to do throughout the organization.
And that's what allows you to build those structures that give you all of those different insights. And again, all of it needs to be tied together with metrics, because we need to measure those processes. We need to measure the impact.
[00:30:20] Mahan Tavakoli: Many of the organizations that I've dealt with traditionally have had a lot of that data in the customer journey that you talked about in the employee journey and part of customer journey., in the sales process, there are tons of data that is sitting on the sales process. That's right. Could lead to tremendous potential impact for the organization.
[00:30:43] Seth Earley: That's right. That's right. Prioritizing, the targets, prioritizing who has a propensity to buy. Having the right intervention, being able to understand where they're in their journey stage, understanding their mental model, and understanding their digital bodyline.
How are they interacting? What are they responding to? There's a model of the customer. That can be as complex as the model for product information. And when you understand that and you harvest that from every touchpoint, you get that 360 degree view of the customer.
Then when you have that, you know how to interact with them. There's a lot in between those things, but you can orchestrate that experience once you have that data model, and once you're collecting it, you can act on it and you can activate the insights from that data.
[00:31:29] Mahan Tavakoli: You do a workshop
for organizations to determine where the right application of AI would be.
[00:31:38] Seth Earley: Yes, we do an AI readiness workshop and what that does is it gets everybody on the same page with definitions. Understanding kinda level sets, it's great for executives, it's great for line of business managers.
And what you do is you start understanding where are our opportunities, what part of the business do we want to impact? What are good use cases? What are the types of interventions that we want to have? And then you can build a roadmap from that. And you can really quickly zero in on some great opportunities for the organization.
And then you can start laying those. And then understanding what needs to be put in place. I don't consider us an AI company. I consider us an IA company, we help organizations with the information architecture and the process analysis. And what we'll do is we'll help guide the organization in terms of where they should spend their energy, where they should focus their resources, where they can have the greatest measurable impact and what they need to do to prepare for that AI powered future.
[00:32:37] Mahan Tavakoli: And that is really important because there is no AI without ia, so we need to get that ia.
Yes, I've
[00:32:43] Seth Earley: heard that. I've heard that. In fact, it's funny. Roed from IBM used to system with the World Economic Forum when she was ceo and people asked her, why is AI having hard time? She said there's a funny saying in our industry there's no AI without ia. She explained it. Tom Davenport was the one who said, GU Romney stole your line.
So IBM's AppD, I really like them to give me credit for it. It is on the inter web. You can't find anybody referencing it before. I wrote that paper, but who knows? I'll give you credit for it, Seth.,
[00:33:13] Mahan Tavakoli: I'm curious you wrote the book a couple of years back, would love to know, in what ways has AI met your expectations? Where is it Yeah. Ahead of where you expected it to be? Yeah. And what do you see over the next couple of years coming with ai?
[00:33:30] Seth Earley: Where I see things going in the next couple years, I really think there's a lot of work that still needs to be done in the foundation and the basics on the fundamentals. And we're gonna see more generative ai, we're gonna see more automation, we're going to see increasing levels of personalization.
That is true personalization. Not just basic, shopping cart recommendations, but we'll start to see people really monitoring and acting on the customer's digital body language. And that is gonna require those approaches. It's gonna require componentized content. So you can recombine the messaging, the different hero image that can call to action, different value proposition, the different target,
and those can be optimized using machine learning, but you need the building blocks, so we'll see more of that. I think the pendulum is gonna shift a little bit. You have these large language models. That a lot of these technologies are built on, chat. G p t is a large language model. , but some of these that are applied to the enterprise are too big, they're too general. So you're gonna see much more of a need to build specialized language models.
That is the ia, that is the enterprise ontology. Think of it this way. If you use something that is generally available to everybody, where's your competitive advantage, because everybody has it, right? But standardization is for efficiency. Differentiation is for competitive advantage. So you'll start to see organizations increasingly focus on building their ontology, their understanding of their customers, their understanding of their knowledge infrastructure, their knowledge architecture, their knowledge ecosystem.
And that's what you compete on. You compete on knowledge of the customer, knowledge of the problems, knowledge of the solutions, knowledge of where you fit in the competitive landscape, knowledge of how to get to those customers, knowledge of what's gonna resonate with them. That's what organizations build their value off.
And the only way to differentiate is to be able to capture and codify that and to be able to leverage it at scale. And the only way to do that is to build your own language model that's going to be about your products, your services, your customers, your employees, and your relationships with them.
That's what you're going to see in the future. And that's where organizations are gonna truly see a lot of value. And then the ones that don't do that are going to be left behind because the organizations that have that will be able to provide better customer service, more personalized at lower cost,
and at a higher value to the customer. And so once that. Train leaves a station, it will be hard. That's when you are really gonna be disrupted by those companies that are doing those things. That other company I mention is seven, seven to 10 years ahead of the market and they are reaping the rewards of that and it's gonna be hard for others to catch up with. There
[00:36:23] Mahan Tavakoli: is tremendous potential in it. For leaders who are not necessarily tech leaders, but aware of the need for understanding ai, the data and moving their organizations forward, what are some resources in addition to your book that you recommend, Seth? We have
[00:36:45] Seth Earley: a ton of stuff on our website.
If you go in the insight section, there's just hundreds of articles that I've written over the years and they're still valid, cause they still cover the basics. There's some great stuff from Jared Spools Company, and I think it's u i e, user Interface Engineering. But they also have courses that can retrain people and train people in user experience and usability and information architecture. I think that's a great resource. There's the Marketing AI Institute, which is an organization that has great value, great information and has a really nice conference.
There's a C M S wire, which is been around for a very long time and they have a huge number of articles from various authors. There's customer Think, which is another website. These are all places where I've either, contributed or worked with them or done stuff with them.
So those are some that you should definitely look into, but they're at various levels of detail, there's some that are a little more deep in terms of. The blocking and tackling, and there's others that are more strategic but those are some good resources to check out. And of course, early.com, e a r l e y.
Don't forget the evil for the y. And just check out the insight section. And we have webinars that we recorded. So those are all great resources and there's no charge.
[00:37:59] Mahan Tavakoli: Those are great recommendations. I appreciate it. And most especially your own website. Also, Seth, as you mentioned, there's a lot of great content and your podcast as well.
[00:38:14] Seth Earley: Oh, thank you. That's right. There's early AI is what it's called, E A R L E Y, and that's also on the website.
So we get some great guests on that.
[00:38:22] Mahan Tavakoli: Lots of outstanding thoughts and perspectives, most especially because as you mentioned, there are the business outcomes that AI can help with the right dataset, help the organizations truly transform toward those business outcomes when measured well, it's not just an experimentation for the fun of it.
It is something that can be transformative for the organization and your insights, your understanding and the way you communicate it can help leaders incorporate that thinking in their organization. So I really appreciate all the insights you have shared through the different platforms, including this conversation.
Thank you so much, Seth.
[00:39:04] Seth Earley: Thank you for the kind words, and thank you for having me. I've had a lot of fun working with you, so this has been great.